Test-Time Alignment of Diffusion Models without reward over-optimization
Best AI papers explained - A podcast by Enoch H. Kang - Fridays
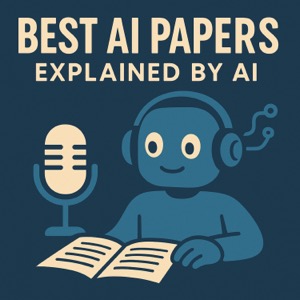
Categories:
This text introduces Diffusion Alignment as Sampling (DAS), a novel approach for aligning diffusion models with desired characteristics by treating the problem as sampling from a reward-aligned distribution. DAS utilizes a Sequential Monte Carlo (SMC) framework enhanced with tempering and a specially designed proposal distribution to efficiently generate high-reward samples without requiring additional training of the diffusion model. The method demonstrates superiority over existing guidance and fine-tuning techniques in single and multi-objective reward optimization, cross-reward generalization, diversity preservation, and online black-box optimization. Theoretical analysis supports the benefits of tempering for improving sample efficiency and mitigating issues like over-optimization and manifold deviation. Experiments across various tasks, including image generation with different reward functions and complex multimodal distributions, validate the practical effectiveness and broad applicability of DAS.