Test-Time Preference Optimization: On-the-Fly Alignment via Iterative Textual Feedback
Best AI papers explained - A podcast by Enoch H. Kang - Fridays
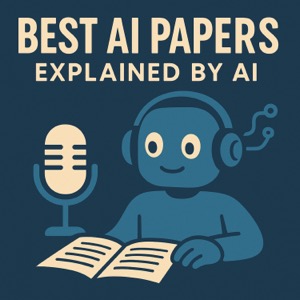
Categories:
This academic paper introduces Test-Time Preference Optimization (TPO), a novel method for improving the performance and safety alignment of large language models during inference without altering their core parameters. Unlike traditional alignment techniques that modify the model during training using numerical gradients, TPO leverages the model's own abilities to interpret numerical reward signals into textual feedback, iteratively refining generated responses through text-based critiques and suggestions. The paper demonstrates that TPO can effectively enhance both unaligned and already aligned models on various benchmarks, achieving results comparable to or exceeding models aligned through more computationally expensive training methods. Furthermore, TPO improves the inference stability of models by concentrating probability mass towards higher-quality outputs, representing a more efficient and flexible approach to model alignment.