Tina: Tiny LoRA Reasoning Models
Best AI papers explained - A podcast by Enoch H. Kang - Tuesdays
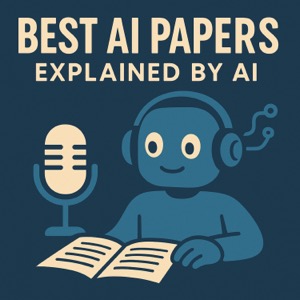
Categories:
We discuss Tina, a family of efficient reasoning models achieved by applying Low-Rank Adaptation (LoRA) during reinforcement learning to a small 1.5B parameter language model. This approach demonstrates that strong reasoning performance, competitive with larger models, can be attained with significantly reduced computational costs. The authors explore the effectiveness of this minimalist strategy across various reasoning tasks and ablation studies, hypothesizing that LoRA facilitates rapid adaptation to the structural format of reasoning. Ultimately, Tina aims to democratize the development of reasoning models by showcasing a highly cost-effective and reproducible methodology, with all code and models being open-sourced.