Tractable Multi-Agent Reinforcement Learning through Behavioral Economics
Best AI papers explained - A podcast by Enoch H. Kang - Fridays
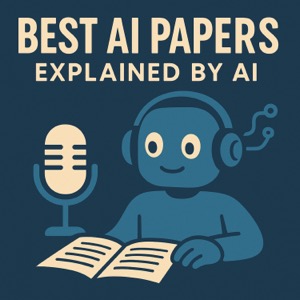
Categories:
This research addresses the difficulty of computing stable outcomes in multi-agent reinforcement learning by incorporating principles from behavioral economics. The authors introduce risk aversion and bounded rationality into game theory, leading to a new solution concept called risk-averse quantal response equilibrium (RQE). They demonstrate that RQE can be computationally tractable in various game settings, unlike traditional Nash equilibria, and that this approach aligns with observed human behavior in experimental games. Furthermore, the work extends this concept to dynamic Markov games, providing algorithms and theoretical guarantees for computing RQE, even in scenarios with unknown game dynamics, thus paving the way for more practical multi-agent learning algorithms.