Trust or Escalate: LLM Judges with Provable Guarantees for Human Agreement
Best AI papers explained - A podcast by Enoch H. Kang - Tuesdays
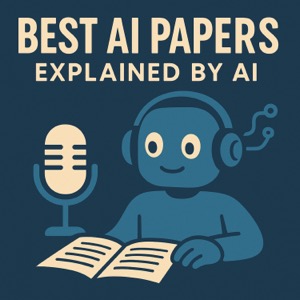
Categories:
This research introduces a new method called Cascaded Selective Evaluation to improve the reliability of using large language models (LLMs) as judges for evaluating text generation. This approach uses a confidence estimation technique called Simulated Annotators to determine when an LLM's judgment is likely to align with human preferences. By selectively trusting LLMs based on their confidence and escalating to stronger models only when needed, the framework provides a provable guarantee of human agreement while also being more cost-effective than solely relying on the most powerful LLMs. Experimental results across different evaluation tasks demonstrate that this method achieves high human agreement with increased efficiency, even outperforming top-tier models in certain scenarios.