Why Do Multi-Agent LLM Systems Fail?
Best AI papers explained - A podcast by Enoch H. Kang - Fridays
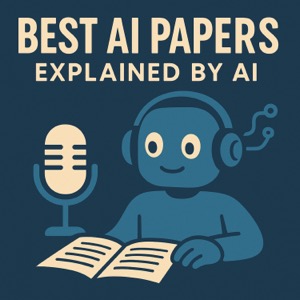
Categories:
This paper addresses the underperformance of multi-agent large language model systems (MAS) compared to single-agent frameworks. To understand this discrepancy, the authors introduce MAST (Multi-Agent System Failure Taxonomy), an empirically developed classification of MAS failures. Through the analysis of several MAS frameworks and diverse tasks, they identified 14 distinct failure modes categorized into specification issues, inter-agent misalignment, and task verification. The research also presents an LLM-as-a-judge pipeline for automated evaluation using MAST and demonstrates its utility through case studies, revealing that system design flaws, rather than just LLM limitations, often cause failures. The authors conclude by emphasizing the need for structural improvements in MAS design and offer their dataset and evaluation tools to facilitate further research.