#120 Applying ML Learnings - Especially About Drift - To Data Mesh - Interview w/ Elena Samuylova
Data Mesh Radio - A podcast by Data as a Product Podcast Network
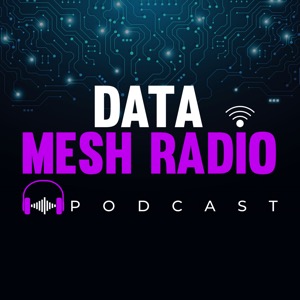
Categories:
Sign up for Data Mesh Understanding's free roundtable and introduction programs here: https://landing.datameshunderstanding.com/Please Rate and Review us on your podcast app of choice!If you want to be a guest or give feedback (suggestions for topics, comments, etc.), please see hereEpisode list and links to all available episode transcripts here.Provided as a free resource by Data Mesh Understanding / Scott Hirleman. Get in touch with Scott on LinkedIn if you want to chat data mesh.Transcript for this episode (link) provided by Starburst. See their Data Mesh Summit recordings here and their great data mesh resource center here. You can download their Data Mesh for Dummies (info gated) here.Elena's LinkedIn: https://www.linkedin.com/in/elenasamuylova/Evidently AI on GitHub: https://github.com/evidentlyai/evidentlyEvidently AI Blog: https://evidentlyai.com/blogIn this episode, Scott interviewed Elena Samuylova, Co-Founder and CEO at the ML model monitoring company - and open source project - Evidently AI. This write-up is quite a bit different from other recent episode write-ups. Scott has added a lot of color on not just what was said but how it could apply to data and analytics work, especially for data mesh. Some key takeaways/thoughts this time specifically from Scott's point of view:A good rule of software that applies to ML and data, especially mesh data products: "If you build it, it will break." Set yourself up to react to that.Maintenance may not be "sexy" but it's probably the most crucial aspect of ML and data in general. It's very easy to create a data asset and move on. But doing the work to maintain is really treating things like a product.ML models are inherently expected to degrade. When they degrade - for a number of reasons - they must be retrained or replaced. Similarly, on the mesh data product side, we need to think about monitoring for degradation to figure out if they are still valuable or how to increase value.Data drift - changes in the information input into your model, e.g. a new prospect base - can cause a model to not perform well, especially against this new segment of prospects. That data drift detection could actually be a very...