#46 Designing a Data Literacy Approach for Data Engineers - Interview w/ Dan Sullivan
Data Mesh Radio - A podcast by Data as a Product Podcast Network
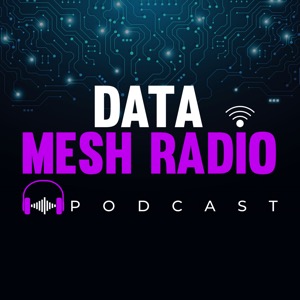
Categories:
Sign up for Data Mesh Understanding's free roundtable and introduction programs here: https://landing.datameshunderstanding.com/Please Rate and Review us on your podcast app of choice!If you want to be a guest or give feedback (suggestions for topics, comments, etc.), please see hereEpisode list and links to all available episode transcripts here.Provided as a free resource by Data Mesh Understanding / Scott Hirleman. Get in touch with Scott on LinkedIn if you want to chat data mesh.Transcript for this episode (link) provided by Starburst. See their Data Mesh Summit recordings here and their great data mesh resource center here.Dan's LinkedIn: https://www.linkedin.com/in/dansullivanpdx/Dan's Email: dan.sullivan at 4mile.ioIn this episode, Scott interviewed Dan Sullivan, Principal Data Architect at 4 Mile Analytics. A key point Dan brought up is tech debt around data. Taking on tech debt should ALWAYS be a very conscious choice. But the way most organizations work with data, it is much more of an unconscious choice, especially by data producers, who are taking on debt that the data engineering teams will have to pay down. We need to find ways to deliver value quickly but with discipline.Zhamak has mentioned in a few talks that data engineers soon may not exist in orgs deploying data mesh. Dan actually somewhat agrees that data engineering will change a lot as right now, there is a big rush to build out the initial iterations of data products (the industry definition). Going forward, Dan thinks there will be a need for data engineers that can really understand consumer needs and build the interactions, e.g. the SDKs, to leverage data.Dan has 3 key pillars for driving data literacy for data engineers are domain knowledge, learning, and collaboration. Data engineers should pair with business people to acquire domain knowledge, they should be given the opportunity to spend time doing things like online training to learn, and they should collaborate across the organization instead of just being ticket tacklers.Per Dan, not all data engineers are the same depending on background - some come from a data analyst/data science background but many come from a software engineering background. So we can't treat training all data engineers as if it's the same. But we do need them to have a well-rounded background. A big need is for them to understand more about the data consumers and/or the producers so embedding them in the domains can really help.For driving buy-in with data engineers, Dan points to the problems typically being around incentives. Data engineering is often hampered by organizational issues and a lack of clear direction. So if you can tackle those, you can often win over DEs. In any organization but especially in one implementing data mesh, standards, protocols, and contracts are all very important....