#50 Evaluating if Data Mesh is a Fit; Team Structures; and WTF is Federated Computational Governance - Interview w/ Marius Ingjer
Data Mesh Radio - A podcast by Data as a Product Podcast Network
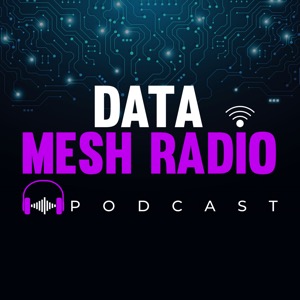
Categories:
Sign up for Data Mesh Understanding's free roundtable and introduction programs here: https://landing.datameshunderstanding.com/Please Rate and Review us on your podcast app of choice!If you want to be a guest or give feedback (suggestions for topics, comments, etc.), please see hereEpisode list and links to all available episode transcripts here.Provided as a free resource by Data Mesh Understanding / Scott Hirleman. Get in touch with Scott on LinkedIn if you want to chat data mesh.Transcript for this episode (link) provided by Starburst. See their Data Mesh Summit recordings here and their great data mesh resource center hereMarius' LinkedIn: https://www.linkedin.com/in/marius-ingjer-a155313/Marius' Medium: https://medium.com/@marius.ingjerIn this episode, Scott interviewed Marius Ingjer, Co-founder and Senior Consultant at Knirkefritt AS. Marius has been working with a few clients on implementing data mesh. They covered 3 distinct topics: evaluating if data mesh is a fit for your organization, team structure challenges in data mesh or data mesh-like implementations, and a simplified definition of federated computational governance.Evaluating if data mesh is right for you:To start, Marius provided a list of evaluation questions to help you determine if data mesh might be right for you: How many data sets are you producing?What is the lead time to creating a new dataset?How well are your datasets serving your data needs?How many domains do you have?How complex are your domains?How does the team respond to new data requirements?How usable in general is your data?Every company wants to share data well but the centralized data team isn't the bottleneck yet for many. Centralization can add a lot of value until it starts to become more hurtful than helpful and yes, figuring out that point is easier said than done. Centralization of data fights Conway's Law and can become way too much cognitive load so it will eventually become an issue for many organizations.A key question in evaluating if data mesh is a fit: what is the cost of allowing your data processes to fail? Per Marius, the business consequence of failed reports has historically not been that high. But if you are driving business decisions, whether that is ML or just crucial day-to-day decisions on your data, data mesh might become more attractive.Team structures and challenges in data mesh:In general, it's important to understand that implementing data mesh will cause cultural challenges - Marius believes developers generally don't want to ALSO share their data. It's additional work so you have to align incentives, which is far easier said than done. That...