Semantic Search: A Deep Dive Into Vector Databases (with Zain Hasan)
Developer Voices - A podcast by Kris Jenkins - Thursdays
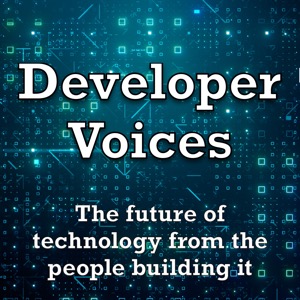
Categories:
As interesting and useful as LLMs (Large Language Models) are proving, they have a severe limitation: they only know about the information they were trained on. If you train it on a snapshot of the internet from 2023, it'll think it's 2023 forever. So what do you do if you want to teach it some new information, but don't want to burn a million AWS credits to get there? In exploring that answer, we dive deep into the world of semantic search, augmented LLMs, and exactly how vector databases bridge that gap from the old dog to the new tricks. Along the way we'll go from an easy trick to teach ChatGPT some new information by hand, all the way down to how vector databases store documents by their meaning, and how they efficiently search through those meanings to give custom, relevant answers to your questions. -- Zain on Twitter: https://twitter.com/zainhasan6 Zain on LinkedIn: https://www.linkedin.com/in/zainhas Kris on Twitter: https://twitter.com/krisajenkins Kris on LinkedIn: https://www.linkedin.com/in/krisjenkins/ HNSW Paper: https://arxiv.org/abs/1603.09320 ImageBind - One Embedding Space To Bind Them All (pdf): https://openaccess.thecvf.com/content/CVPR2023/papers/Girdhar_ImageBind_One_Embedding_Space_To_Bind_Them_All_CVPR_2023_paper.pdf Weaviate: https://weaviate.io/ Source: https://github.com/weaviate/weaviate Examples: https://github.com/weaviate/weaviate-examples Community Links: https://forum.weaviate.io/ and https://weaviate.io/slack -- #vectordb #vectordatabase #semanticsearch #openai #chatgpt #weaviate #knn