Adaptive Networks and Interpretable AI the Future with Kolmogorov Arnold Networks
Digital Innovation in the Era of Generative AI - A podcast by Andrea Viliotti
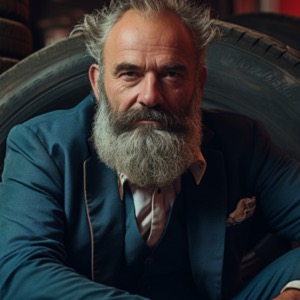
The article introduces Kolmogorov-Arnold Networks (KAN) as an innovative neural network architecture that offers an alternative to traditional Multi-Layer Perceptrons (MLP). KAN are based on the Kolmogorov-Arnold representation theorem and use learnable activation functions on the connections between nodes, rather than on the nodes themselves. This approach gives KAN greater flexibility and precision compared to MLPs, making them particularly suitable for complex scientific and industrial applications. The sources illustrate the advantages of KAN, such as the ability to handle complex data, accuracy in approximations, and ease of interpretation. The architecture of KAN, the training process, and their approximation capabilities are analyzed. The sources also discuss the challenges that still need to be addressed to optimize KAN, such as the search for new activation functions, managing computational efficiency, and integrating with existing machine learning architectures. Ultimately, the sources present KAN as a promising frontier in artificial intelligence, paving the way for new advances in precision, interpretability, and the ability to solve complex problems.