From CoT to Self-Discover the future of digital reasoning in LLMs
Digital Innovation in the Era of Generative AI - A podcast by Andrea Viliotti
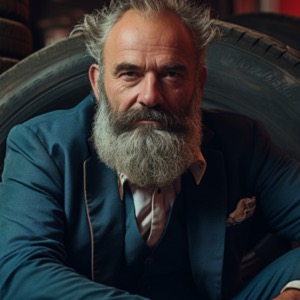
Self-Discover represents an innovative overarching framework for large language models (LLMs) that enables the self-discovery of reasoning structures to tackle complex problems. It utilizes atomic reasoning modules to compose a task-specific thinking framework, enhancing GPT-4 and PaLM 2 on tests like BigBench-Hard and MATH. Compared to Chain of Thought (CoT), Self-Discover improves performance by up to 32%, requiring fewer inference calculations than CoT-Self-Consistency. Applicable to various models, it simulates human reasoning.