Reliability of AI Models. The Paradox of GPT, LLaMA, and BLOOM Models: Advanced Performance, Yet Errors in Simple Tasks
Digital Innovation in the Era of Generative AI - A podcast by Andrea Viliotti
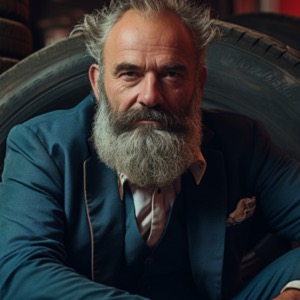
Large language models (LLMs) like GPT and LLaMA are becoming increasingly powerful, yet a recent study has highlighted a concerning paradox: while these models improve at handling complex tasks, they tend to make more errors in simple tasks. The study examines this phenomenon by exploring the relationship between perceived task difficulty and the accuracy of responses, the models' tendency to avoid answering difficult questions, and their sensitivity to variations in question formulations. The findings show that, despite increases in size and optimization, these models are not yet reliable in contexts where precision is critical, particularly in crucial areas such as health, safety, or law. The study suggests a need to rethink development strategies to ensure more reliable accuracy and response stability, moving away from the purely expansive approach that has so far dominated the field of artificial intelligence.