019 - The Non-Technical (Human!) Challenges that Can Impede Great Data Science Solutions
Experiencing Data w/ Brian T. O’Neill (UX for AI Data Products, SAAS Analytics, Data Product Management) - A podcast by Brian T. O’Neill from Designing for Analytics - Tuesdays
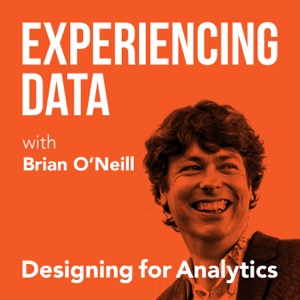
Categories:
Dr. Bob Hayes, will be the first to tell you that he’s a dataphile. Ever since he took a stats course in college in the 80s, Bob’s been hooked on data. Currently, Bob is the Research and Analytics Director at Indigo Slate. He’s also the president of Business over Broadway, a consultancy he founded in 2007.In a past life, Bob served as Chief Research Officer at Appuri and AnalyticsWeek, Chief Customer Officer at TCELab, and a contributing analyst at Gleanster, among many other roles. In today’s episode, Bob and I discuss a recent Kaggle survey that highlighted several key non-technical impediments to effective data science projects. In addition to outlining what those challenges are and exploring potential solutions to them, we also covered: The three key skills successful data science teams have Why improving customer loyalty involves analyzing several metrics, not just one Why Bob feels the scientific method is just as important today as it’s been for hundreds of years The importance of repeatable results How prototyping early can save time and drive adoption of data science projects Bob’s advice on how to move data science projects forward (and one key skill he feels most business leaders lack) The role of the analytics translator Resources and Links: Dr. Bob Hayes on LinkedIn Seeing Theory Calling Bullshit Doctor Bob Hayes on Twitter Business Over Broadway IndigoSlate Quotes from Today’s Episode “I’ve always loved data. I took my first stats course in college over 30 years ago and I was hooked immediately. I love data. Sometimes I introduce myself as a dataholic. I love it.” — Bob “I’m a big fan of just kind of analyzing data, just getting my hands on data, just exploring it. But that can lead you down a path of no return where you’re just analyzing data just to analyze it. What I try to tell my clients is that when you approach a data set, have a problem that you’re trying to solve. The challenge there I think it stems from the fact that a lot of data science teams don’t have a subject matter expert on the team to pose the right questions.” — Bob “The three findings that I found pretty interesting were, number one, a lack of a clear question to be answering or a clear direction to go in with the available data. The second one was that data science results were not used by the business decision makers. And the third one was an inability to integrate findings into the organization’s decision making processes.” — Brian “It makes you wonder,‘if you didn’t have a good problem to solve, maybe that’s why [the findings] didn’t get used in the first place.’” — Brian “That part isn’t so much the math and the science. That’s more the psychology and knowing how people react. Because you’re going to have certain business stakeholders that still want to kind of shoot from the hip and their experience. Their gut tells them something. And sometimes that gut is really informed.” — Brian “If executives are looking at data science and AI as a strategic initiative, it seems really funny to me that someone wouldn’t be saying, ‘What do we get out of this? What are the next steps?’ when the data teams get to the end of a project and just moves on to the next one.” — Brian