080 – How to Measure the Impact of Data Products…and Anything Else with Forecasting and Measurement Expert Doug Hubbard
Experiencing Data w/ Brian T. O’Neill (UX for AI Data Products, SAAS Analytics, Data Product Management) - A podcast by Brian T. O’Neill from Designing for Analytics - Tuesdays
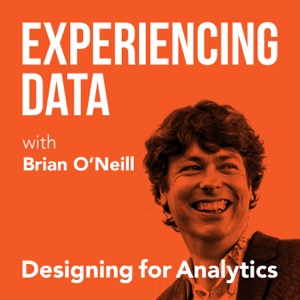
Categories:
Finding it hard to know the value of your data products on the business or your end users? Do you struggle to understand the impact your data science, analytics, or product team is having on the people they serve? Many times, the challenge comes down to figuring out WHAT to measure, and HOW. Clients, users, and customers often don’t even know what the right success or progress metrics are, let alone how to quantify them. Learning how to measure what might seem impossible is a highly valuable skill for leaders who want to track their progress with data—but it’s not all black and white. It’s not always about “more data,” and measurement is also not about “the finite, right answer.” Analytical minds, ready to embrace subjectivity and uncertainty in this episode! In this insightful chat, Doug and I explore examples from his book, How to Measure Anything, and we discuss its applicability to the world of data and data products. From defining trust to identifying cognitive biases in qualitative research, Doug shares how he views the world in ways that we can actually measure. We also discuss the relationship between data and uncertainty, forecasting, and why people who are trying to measure something usually believe they have a lot less data than they really do. Episode Description A discussion about measurement, defining “trust”, and why it is important to collect data in a systematic way. (01:35) Doug explores “concept, object and methods of measurement” - and why most people have more data than they realize when investigating questions. (09:29) Why asking the right questions is more important than “needing to be the expert” - and a look at cognitive biases. (16:46) The Dunning-Kruger effect and how it applies to the way people measure outcomes - and Bob discusses progress metrics vs success metrics and the illusion of cognition. (25:13) How one of the challenges with machine learning also creates valuable skepticism - and the three criteria for experience to convert into learning. (35:35) Quotes from Today’s Episode “Often things like trustworthiness or collaboration, or innovation, or any—all the squishy stuff, they sound hard to measure because they’re actually an umbrella term that bundles a bunch of different things together, and you have to unpack it to figure out what it is you’re talking about. It’s the beginning of all scientific inquiry is to figure out what your terms mean; what question are you even asking?”- Doug Hubbard (@hdr_frm) (02:33) “Another interesting phenomenon about measurement in general and uncertainty, is that it’s in the cases where you have a lot of uncertainty when you don’t need many data points to greatly reduce it. [People] might assume that if [they] have a lot of uncertainty about something, that [they are] going to need a lot of data to offset that uncertainty. Mathematically speaking, just the opposite is true. The more uncertainty you have, the bigger uncertainty reduction you get from the first observation. In other words, if, you know almost nothing, almost anything will tell you something. That’s the way to think of it.”- Doug Hubbard (@hdr_frm) (07:05) “I think one of the big takeaways there that I want my audience to hear is that if we start thinking about when we’re building these solutions, particularly analytics and decision support applications, instead of thinking about it as we’re trying to give the perfect answer here, or the model needs to be as accurate as possible, changing the framing to be, ‘if we went from something like a wild-ass guess, to maybe my experience and my intuition, to some level of data, what we’re doing here is we’re chipping away at the uncertainty, right?’ We’re not trying to go from zero to 100. Zero to 20 may be a substantial improvement if we can just get rid of some of that uncertainty, because no solution will ever predict the future perfectly, so let’s just try to reduce some of that uncertainty.”- Brian T. O’Neill (@rhythmspice) (0