The Data Lakehouse: Brick by Brick, w/ Databricks' Denny Lee
Raw Data By P3 Adaptive - A podcast by P3 Adaptive - Tuesdays
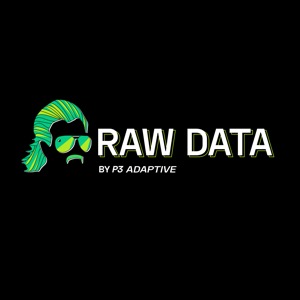
Categories:
We dive into the deep end of the Data Lake on this episode with our guest, Senior Staff Developer Advocate at Databricks, Denny Lee. Denny knows so much about Delta Lake, Apache Spark, Data Lakes, Data Warehouses, and all of the tech that is involved. At one point Rob’s mind gets so blown by something that Denny talks about, his jaw may still be on the floor! Episode Transcript: Rob Collie (00:00:00): Hello friends. Today's guest is Denny Lee. Absolutely one of the most friendly, outgoing, and happiest people that you'll ever hear on this show, that you'll ever hear anywhere, for that matter. And it's a little bit different. This episode, we spend a lot of time focused on technology, which we very often don't do. But the reason why we did is because Denny represents an amazing opportunity for us to explore what's happening like in this parallel universe of data. Most of us are up to our eyeballs in the Microsoft data platform and not even the entire Microsoft data platform, but very specific portions of it like centered on Power BI, for instance. Rob Collie (00:00:45): In the meantime, there's this entire universe of technology running on Linux in the cloud, that if you watch the show Silicon Valley, these are the tools being used by those people on that show. And also by the way, in the real Silicon valley, and to know someone like Denny, who has walked in the same world as us, because he's fully entrenched in this other world, I couldn't resist the opportunity to have him translate a lot of the things from that world for the rest of us. Rob Collie (00:01:16): In the course of this conversation, there's an absolutely jaw dropping realization that hits me that I was completely unaware of. I couldn't believe, I still can't believe that there was an ongoing flaw, an ongoing weakness in Data Lake technology that's only recently being addressed. And by the time we were done recording this, it was clear to me that we need to do it again, because there are so many things left to explore in that same day. We could have at least one more episode like this. So we're definitely going to have him back. So buckle up for a journey into the world of Apache Spark and Hadoop and Data Lakes and Lake Houses and Delta Lakes. Jason and the Argonauts make an appearance. We talk about photon, but most importantly, we talk about why this would be ever relevant to the Power BI crowd. So let's define that world, Denny's world as it, and then let's get into it. Announcer (00:02:22): This is The Raw Data by P3 Adaptive podcast with your host, Rod Collins and your cohost, Thomas Larock. Find out what the experts at P3 Adaptive can do for your business. Just go to P3Adaptive.com. Raw data by P3 Adaptive is data with the human element. Rob Collie (00:02:45): Welcome to the show. Denny Lee, I haven't spoken with you in, gosh, it's coming up probably on 10 years, right? Like it's getting close. Denny Lee (00:02:55): Yeah. It's been a long while. It's been a long time. You ran to the Midwest and I just wanted nothing to do with you. Rob Collie (00:03:01): That's right. I mean, that's the natural reaction from the Seattle tribes. Denny Lee (00:03:05): Oh, absolutely. Yeah, we're bad like that. Yeah. Rob Collie (00:03:08): It's like that scene in Goodfellas where the boss of [inaudible 00:03:11] says, and now I got to turn my back. Denny Lee (00:03:13): Exactly. Yeah. You went over the cascades. I'm done. Rob Collie (00:03:17): That's right. So, but you also seem to have left the other family, the Microsoft family. Denny Lee (00:03:24): That's very true. I did do that, didn't I. It was a long time ago. Rob Collie (00:03:28): This is like one outcast speaking with another. Denny Lee (00:03:31): That's true. That's true. We are outcasts. That's fair. But I mean, I don't think that had necessarily to do with leaving the big ship either though. I think we were just outcasts in general. Rob Collie (00:03:38): That was our role. Denny Lee (00:03:39): It was, yeah. It was all brand for us. Rob Collie (00:03:43): We're not going to spend too much time on history here, but well, we can, but there are a number of things that I do want to know about your origin story. You and I met basically over the internet, even though we were both Microsoft employees at the time- Denny Lee (00:03:58): And on the same campus. Rob Collie (00:03:59): ... And you showed up on my radar when Project Gemini and Power Pivot was actually getting close to like beta and stuff. Right. Denny Lee (00:04:07): That's right. That's right. Rob Collie (00:04:08): And you just materialized. It was like, now it's time to talk about these things publicly. And there was Denny. Denny Lee (00:04:15): Yes. Yes. [inaudible 00:04:17] loud. Rob Collie (00:04:17): Well, look who you're talking to. Denny Lee (00:04:21): Fair enough. Fair enough. Mind you, this is a podcast that I don't think anybody can see anything by the way. You do know that, right? Rob Collie (00:04:25): Yeah, I know. Yeah. They're not recording the video. Denny Lee (00:04:28): Thank you. Rob Collie (00:04:29): So what was your role back then? What got you associated with Power Pivot Project Gemini? Denny Lee (00:04:35): I'll be honest. What associated with, because I was going, "Why in expletive were we doing this?" In fact, because before this, I was on the SQL customer advisory team, I was the BIDW lead. BIDW. I know big, big words and the reason I bring that up is only because we had just announced maybe what, nine months prior, the largest analysis services cube on the planet, which was the Yahoo Cube so that was 20... At the time that was back in what, 2010, 24 terabyte queue built on top of like, I want to say two perabyte, 5,000, [inaudible 00:05:14] cluster. And so at the time that was a pretty big thing. So it's probably even bigger thing now. So whatever, but still the point being like, especially back in 2010, that's pretty huge. And so I'm going like, "Okay. So I just helped build the largest cube on the planet." And so now we're going to focus on this thing, which is this two gigabyte model. And basically my jaw dropped to the floor. Denny Lee (00:05:34): I'm going, "I just helped build the largest cube on the planet and you want me to help build a two gigabyte model? You sure you didn't mix up the T and the G here? Like what, wait, what's going on here? So that's how I got involved. But suffice to say, after talking to you, after talking to Kamala, after talking to some of the other folks, I realized, "Oh, I'm missing the point." I actually missed the whole point altogether about this concept of self-serve BI because, of course, everything before was very much IT based BI. So yes, it makes sense for an IT team to go ahead and build the 24 terabytes. Actually. No, it doesn't. But nevertheless, you don't want to ask your domain expert to basically build a 24 terabyte cubes. That seems like a really bad idea. So yes. Yeah. But that's how you and I connected because I was going like, "Wait, why are we doing this?" And then after being educated by you, realized, "Oh, okay, cool. This is actually a lot cooler than I thought it would be." Rob Collie (00:06:33): It's really interesting to think about it. The irony, right, is that I was thinking about Power Pivot in light of like, holy cow, look at all this data capacity we can put into Excel now. This is just like orders and orders of magnitude explosion in the amount of data that can be addressed by an Excel Pivot Table. To me, it was like science fiction level increase and you're going, "That's it." Denny Lee (00:07:01): Exactly. [crosstalk 00:07:03]. Rob Collie (00:07:05): Now, in fairness, I mean the compression does turn that two gigabytes and that's... The two gigabytes was the limit for file sizes in Office, but more specifically in SharePoint, right? I think it was the SharePoint limits. I wonder if that's even relevant today, but at the time it was super relevant, but yeah, the two gigabyte file size limit, even when compressed, might've been the equivalent of a 30 or 40 gigabyte normal cube, but you were still dealing with a different terabyte model. That's neat. Wait, this is so small. No, no. It's huge, trust us. Yeah. So you are one of the people who could write the old MDX. Denny Lee (00:07:48): That's right. Now we're hitting on the fact that Rob, Tom and I are old people. We're not talking about markdown extensions, all [crosstalk 00:07:55] framework. We're actually talking about MDX as the multi-dimensional expression. Does anybody still use it? Rob Collie (00:08:03): I think it's still used. Yeah. Denny Lee (00:08:04): Okay. Cool. Actually I have no idea- Rob Collie (00:08:06): But it's definitely been, in terms of popularity, it has been radically eclipsed by DAX. I mean even most of, if not all, but most of the former MDX celebrities now spend more time as DAX celebrities. Denny Lee (00:08:22): Do you want to mention names? Rob Collie (00:08:24): We've had some of them on the show, right? We've had Chris Webb, right? Okay. We haven't had the Italians. Denny Lee (00:08:29): Why not? Rob Collie (00:08:30): Well, I think we- Denny Lee (00:08:30): Alberto... Those guys are awesome. Rob Collie (00:08:33): I think we're going to, for sure. I mean, that's going to happen. Our goal is to eventually have like all 10 people who could write MDX back in the day, have them all on the show. We've had plenty of guests on the show where we talk about the origin stories of Power BI and all of that. Not that we couldn't talk about that. We absolutely could, but I think you represent an opportunity to talk about some incredibly different things that are happening today, things that are especially, I think a lot of people listening from the Microsoft data platform crowd might have less experience with a number of the things that you're deeply familiar with these days. And some of them do have experience with it. It's a very interesting landscape these days, in terms of technology like dogs and cats living together, mass hysteria, like from the Ghostbusters. Rob Collie (00:09:18): It's crazy how much overlap there is between different platforms. You can be a Microsoft centric shop and still utilize tons of Linux-based cloud services. And so I know what you're working on today, but let's pretend that I don't. Where are you working today, Danny? What are you doing? What are you up to? Denny Lee (00:09:38): I'm actually a developer advocate at Databricks and so the company Databricks itself was created by the founders of the Apache Spark project, which we're obviously still very active in. The folks behind projects like... And including Apache Spark and the recently [QUADS 00:09:57] project, which was to include the pandas API directly into Spark, but also things like MLflow for machine learning for Delta Lake, which is to bring transactions actually into a Data Lake, projects like that. That's what I've been involved with actually after all these years. Denny Lee (00:10:10): And just to put a little bit of the origin story back in just for a second, this actually all started because of that Yahoo cube. So what happened was that after I helped build the largest cube on the planet with Dave [Mariani 00:10:23] and Co just a shout out to Dave, what happened was that afterwards I was having regular conversations still as a Microsoft guy, right with Yahoo but we invariably went to like, "Wait, we don't want to process this cube anymore," because it would take the entire weekend to process a quarters worth of data. And if we ever need to reprocess all of it, that was literally two weeks offline. Sort of sucky. That's the technical term. Rob Collie (00:10:46): Had to be a technical term. Denny Lee (00:10:49): [crosstalk 00:10:49]Yeah, suck it. So what happened was that we were thinking, "What can we do to solve this problem?" And so we ended up both separately coming to the same conclusion that we were using Spark. So everything in terms of what I did afterwards, which I was part of the nine person team that created what now is known as HDInsight for project Isotope and then eventually joined Databricks was actually all from the fact that I was doing Spark back then, shortly after helping create the largest cube on the planet because of the fact we're going, "We don't want to move that much data anymore." Rob Collie (00:11:21): All right. Let's back up. That was a lot of names of technologies that went by there. It was a blur. Okay. So the founders of Databricks originally created Apache Spark? Denny Lee (00:11:31): Correct. Rob Collie (00:11:32): All right. What is Apache Spark? Denny Lee (00:11:35): Apache Spark is a distributed framework, in memory framework that allows you to process massively large amounts of data across multiple notes, notes servers, computers, things of that nature in a nutshell. Yeah. Rob Collie (00:11:49): So, that's what it does, but in terms of the market need, what market need did it fill? You had this kind of problem and then Apache Spark came along and you're like, "Oh my God." Denny Lee (00:11:58): The concept is, especially back then, the idea of web analytics. It was started with the idea that I needed to understand massive amounts of web data. Initially it was just understanding things like events and stuff. But then of course it quickly dovetailed to advertising, right? I need to understand were my advertising campaigns effective, except I have these massive amount of logs to deal with. In fact, that's what the Yahoo cube was. It was basically the display hats within Yahoo. Could they actually build solid campaigns for display ads on the Yahoo website? Well then what invariably happens is that this isn't just a Yahoo problem. This this is anybody that's doing anything remotely online that they had the same problem. And so what why became what now is considered the de facto big data platform is because of the fact that lots of companies, whether we're talking to the internet scale companies, or even what now are traditional companies, traditional enterprises, when it came down to that, they had a lot of data that was semi-structured or unstructured, as opposed to beautiful flat [crosstalk 00:13:03]. Denny Lee (00:13:03): What you actually had was basically the semi-structured, unstructured key value pairs, [jfonts 00:13:08], all these other myriad of data formats that you actually had to process. And so, because you're trying to process all this data, what it came down to is you need a framework that was flexible enough to figure out how to process all that data. And so in the past, we would to say, "Hey, just check into the database or I mean we share the database and we could [inaudible 00:13:27] it. But the data itself wasn't even in a format that was easy to structure in first place. Rob Collie (00:13:32): Let's start there. The semi-structured and unstructured data revolution. We already had this problem before the internet, right before everybody went digital. But it really made it mainstream. The most obvious example is people like being in Google and Yahoo, these search engines, right? Them going out and indexing and essentially attempting to store the entire contents of the internet so that they can have a search engine. Imagine trying to decompose the contents of a webpage on a website, into a database friendly format. You could spend years on it just to get like one or two websites schema designed to fit... It would absorb one or two websites. And then the world's third website comes along and it doesn't fit. Denny Lee (00:14:19): Exactly. Rob Collie (00:14:19): ... what you designed. And so I've actually, in terms of storage, the whole Hadoop style revolution of storage, I think is awesome, without any reservation. The whole notion of data warehousing, if you just take the words at face value, don't lose anything. And it was very, very, very expensive to use SQL as your way of not losing anything. And so these semi-structured stores were much more like, om, nom, nom, garbage cans. You could just feed them anything and they would keep it. Denny Lee (00:14:49): That's right. Rob Collie (00:14:50): So then we get to the point where, oh, we actually want to go read some of that stuff. Denny Lee (00:14:54): Ah. There we go. Yes. Rob Collie (00:14:55): We don't want someone to store it. We want to be able to like, I don't know, maybe access it again later and do something with it- Denny Lee (00:15:00): Even get some actual value out of it. Rob Collie (00:15:02): Yeah. I mean, is that where Spark comes in? Is at that point? Denny Lee (00:15:06): Absolutely. So we would first start with Hadoop and so the context, if you want to think of it this way, is that because exactly to your point, I could build a functional program with MapReduce to allow me to have the maximum flexibility to basically... Because everybody likes writing in Java, I'm being very sarcastic by the way. Okay. Very, very sarcastic. You can't tell if you don't know me well, but yes. So I want to really call that out. So you love writing Java. You want to write the MapReduce code, but it does give you a massive amount of flexibility on how to parcel these logs and it's a solid framework. So while you wouldn't get the query back quickly, you could get the query back. And so the context is more like, if you're talking about like, especially at the time, you're talking about terabytes of data, right? Denny Lee (00:15:52): The time it would take for me to structure it, put into a database, organize it, realize the Sparks call wasn't working, I realized that I forgot variable A, B, C, and all these other things that you would iterate, especially with the classic waterfall model. By the time we did it, it's like 6, 7, 8 months later, if we're lucky. And then if you had a large enough server. There's all of these ifs. And so what happened with the concept of [inaudible 00:16:15] was like, "Okay, I don't care. It's distributed across these 8, 10, 20 commodity nodes. I didn't need any special server. I run the query, might take two weeks but the query would come back and I would get the result. And the people were like, "Well, why would you want to wait two weeks?" I'm like, "Well, we'll think about it from two ways. One, do I want to wait two weeks or do I want to wait eight months? Number one. Denny Lee (00:16:40): Number two. Yes, it's two weeks but then I can even figure out whether I even needed the data in the first place, right? Rob Collie (00:16:46): That's right. Denny Lee (00:16:47): And so how Spark gets added on top of this is saying, well, we can take the same concept as Hadoop, except do it on any storage, number one, you work specifically to Hadoop, you could do it on any storage, number one. And number two, it will be significantly faster because we could actually put a lot of the stuff... Stuff, a technical term into memory. So I could process the data in memory, as opposed to just going ahead and basically being limited four or eight gigabyte, especially with the older JVMs, right, limited to that much memory to do the processing. Rob Collie (00:17:20): I have a hyper simplified view of all of this, which took me a long time to come around to, which is the old way, was to look at a bunch of data and figure out how to store it in rectangles. And that's very, very, very, very difficult, labor intensive. That's the six, seven months, if you're lucky. And by the way, rule number two is you're never lucky. Denny Lee (00:17:41): Exactly. Rob Collie (00:17:43): So six or seven months is what this project is specked to be at the beginning. And it never comes in on time. Denny Lee (00:17:48): We don't hit that target anyways. Exactly. Rob Collie (00:17:50): And then the world changes and all of your rectangular storage needs to be rethought. Right? Okay. So pre-rectangle-ing the data to store it ends up being just a losing battle. Now analysis, I like to think of analysis. Analysis is always rectangle based. When you go to analyze something, you're going to be looking at the things that these different websites have in common. You always are going to be extracting rectangles to perform your analysis. I loved your description of like, okay, we can take six, seven months wink, wink to store it as rectangles. And then we get fast rectangle retrieval. We think we hope, Denny Lee (00:18:26): We hope. Rob Collie (00:18:26): We probably did not anticipate the right rectangles or we can delay that. We can delay the rectangularization. Store it really easily and cheaply by the way, quickly, easily cheaply. And later when we're fully informed about what rectangles we need, that's when the rectangle work happens and even in the old days, when all we had was Hadoop's original storage engine, two weeks, yeah, I can see that leaving a mark at runtime. That's also what we call a sucky. We call that slow in the tech universe, but it's still better than the six or seven actually 14, 20 months from before. Okay. So Spark walks into that and through some incredibly hocus-pocus magic, just brings fast queries, essentially fast rectangle extraction to that world where you still don't have to pre-rectangle. You still get all the benefits of the unstructured cheap commodity storage, but now you don't have to wait two weeks for it to run. Denny Lee (00:19:25): Right. So if you remember our old Hadoop hive days, we would rally around the benefits of schema on read, right and don't get me wrong. I can go on for hours about that's not quite right. Rob Collie (00:19:34): You're giving me way too much credit right now. I've never touched Hadoop. I'm aware of it and we use Data Lake storage at our company and all that kind of stuff. Think of it this way. I have a historians point of view, a technical historian's point of view on this stuff. But like you start talking about, "Yeah, you remember back in our days and we were like sling..." No, I can play along, but it would feel inauthentic. Denny Lee (00:19:56): No, fair enough. So from the audience perspective, the idea of schema on read in essence using Rob's analogy would be like, you store a bunch of circles, triangles, stars. That's what's in your cheap storage and the idea of schema on read at the time that you run your query at runtime, I will then generate the rectangles based off of the circles and squares and stars that I have in my storage. Rob Collie (00:20:20): Oh, okay. I actually misheard you as well, which I thought you said schema and read. Schema on read. Denny Lee (00:20:27): Yes. Rob Collie (00:20:27): That's awesome. I wasn't even aware of that term, but now that you say it, yeah. That's runtime rectangle. Rectangle when we go to query. Okay, awesome. There was the technical term for- Denny Lee (00:20:37): Yes, there is a technical term for runtime rectangles. That's correct. Rob Collie (00:20:40): I feel so incredibly validated. I will effortlessly slip schema on read into conversation. Denny Lee (00:20:49): Perfect. So, but I'm going to go with the analogy. So in other words, now that we generate runtime rectangles, the whole premise of it was that with Spark we could generate those runtime rectangles significantly faster and get the results to people significantly faster than before with Hadoop. And so that's why Spark became popular. And the irony of it all was that that actually wasn't its original design. Its actual design was actually around machine learning, which is not even runtime rectangles. Now it's runtime erase. So like Vector, so it's a completely different thing, but what ultimately got it to become popular wasn't the runtime arrays of vectors? It was actually the runtime rectangles so people could actually say, "Oh, you mean I don't have to wait two weeks for the query. I can get that query down in a couple of hours?" "Yeah." "Oh, okay. Well then let me go do that." Rob Collie (00:21:39): So that's it, right? Miller time. We're done. That's it. That's the end of the technology story. We don't need [crosstalk 00:21:43] Denny Lee (00:21:43): Yeah, we're done. That's it? There's nothing else there. Thomas Larock (00:21:47): We've hit the peak. Denny Lee (00:21:48): Yeah, that's it. We've hit the peak. Rob Collie (00:21:50): This is the end of history. Right. Denny Lee (00:21:52): Yeah, this is it. Thomas Larock (00:21:54): We're at the end of the universe. Denny Lee (00:21:55): But I'm sure as we all know, the curve, the Gartner hype cycle and everything else for that matter, that's not quite the case. And what's interesting is that especially considering all of us were old database folks or at least very database adjacent, even if we're not database specific. One of the things we realized about this runtime rectangle or the schema on read concept was wait, garbage in, garbage out. Went out of your way to store all of this data. You should do that. In some cases you really do have to leave it in whatever state you get it. Because like whatever the query for the rest API, whatever [inaudible 00:22:36] packet protocol, whatever, whatever format. Sometimes you don't have a choice and that's fine. I'm not against that idea because the context is, especially back in 2011, 2012, we were using statistics like the amount of data generated in the last year was more than the amount of data generated in all of history before that. Thomas Larock (00:22:57): You know that's a bullshit statement, right? Denny Lee (00:22:58): Whether it's a bullshit statement is actually irrelevant to me because the context is not wrong. The reason that statement came up was actually because machines were generating the data now. So since machines are generating the data, the problem is that we don't actually have people in the way of the data being generated. So it's going to be a heck of a lot more than any organic person involved to be able to make sense of that. Rob Collie (00:23:22): I love that exchange there, right? The amount of data generated in this timeframe is more than that timeframe. Tom says, "You know that's a bullshit statement." Then he says, "Hey, do not let the truth get in the way of a good concept." Denny Lee (00:23:33): Exactly. Do not. Do not do not. It's okay. Thomas Larock (00:23:39): That doesn't matter. What I just said doesn't matter. Denny Lee (00:23:42): Exactly to your point though. That's the whole point. It doesn't matter what the statement really is in this case. What really matters is the fact that there is that much generated data. That's what it boils down to. Right? It doesn't matter what the marketing people said. Businesses still had a problem where they had all of this data not structured coming in. And so now the problem is, it's mostly noise. It's mostly garbage and you're going to need time to figure out what's actually useful and what's not. You can automate to your heart's content and so that means, okay, sure, I've processed part of the data to figure that out. But the reality is there's always new devalues, new attributes, new whatever, coming in. At least if you're successful, there's new something coming in. And if there's no something coming in, whatever format that is, you're going to have to figure out what to do with it. Denny Lee (00:24:39): And so at some point, especially when you take into account of things like streaming systems, where basically just data is constantly coming through and it's not like batch oriented at all where basically data's coming through. Multiple streams that ultimately you want to place into a table. What does it imply? It implies that I actually want a rectangle, a structure of some type at the closest point to where the data resides right from the get-go. Because what I want, isn't all of the key value stores. What I want is all the information, but there's a difference between information and data or if you want to go the other way, there's a difference between noise and data. So whatever way you want to phrase it, I'm cool either way with that too. But the fact is the vast majority of what's coming in is noise. You got to extract something out of it. Denny Lee (00:25:32): And then that's where the sticky, coil mining analogies kick in. And again, I'm not going to play with that one, but the point is what it boils down to is that that means I need some structure to what I'm doing, flexibility still. So if I need to change the structure, I can do that, but I need some form of structure. And so what's interesting about this day and age, especially in this day of like we're using Spark as the primary data processing framework, is that there are like, I want to talk about Delta Lake, but as a call-out to other projects, there are other, projects like [inaudible 00:26:01], there's other projects like Apache Iceberg, right? And I'm going to talk about Delta Lake, but we all came roughly the same time with the exact same conclusion. We've been letting so much garbage into our data, we need some form of structure around it so we can actually figure out what's the valuable bits. Rob Collie (00:26:18): It seems like a really hard problem. I mean, even like without technology in the way one person's trash is another person's treasurer. Denny Lee (00:26:25): Exactly. Rob Collie (00:26:26): What's considered trash today is treasure tomorrow. And if I wanted to be really snarky, I'd be like, "Are you saying that we need to go back to rectangular storage in the first place?" So it's back to SQL after all? Denny Lee (00:26:40): Almost. In fact that's exactly what I'm saying. What I'm saying is that I want to get the rectangles as close to the storage, but not actually the storage itself. Okay. The reason I want to get as close to is because of exactly what you said, because maybe today, the stars in my circle, star, square analogy are what's important and then need to be converted into rectangles, but I don't need the circles and I don't need the triangles. Later on, I realize maybe I need the triangles or some of them, not all the triangles. And later on, I may need... You know, forget about the triangles altogether. Let's just put the circles in and whatever else. But the context is exactly that. So you want structured storage as close to the storage, as you can ,without actually going ahead and messing with your input systems. Because typically there's two reasons why you can't mess with it. Denny Lee (00:27:29): One is because you're not controlling it, right? If you're hitting a source system, you're hitting a REST API, it is what it is. That's the source system. So you're going to get whatever it is. And so in order to ensure that you've [inaudible 00:27:42] and you've kept it as close to the original value as possible, your job is basically if it's a REST API call, grab the JSON packet, chuck it down to disk as fast as you can so that way... And validate for that matter, that the JSON's fully formed. So that way, okay, got it. This is the correct thing that also [inaudible 00:27:59] store, but now once you've done that, you can say, "Okay, well out of this JSON packet, I actually only need six of the columns. Rob Collie (00:28:04): Are we talking about dynamic structured caching? Denny Lee (00:28:09): No. Good call, but no, it's not. First of all, there's actually two problems. One is dynamic and one's caching. Thomas Larock (00:28:15): You're right. There's a difference between information and the data. I want to point out that all information is data, but not all data is information, right? [crosstalk 00:28:26] and we can talk about busting your stones about more data's being created because Stephen Hawking would tell you that information is neither created or lost in the universe. It's all around us. Nothing's ever been created or destroyed. No information could be lost, otherwise there's a problem with the universe. So when people talk about what they're really talking about is they're just able to collect more. It's always been there. They just never had the ability to go and get it so easily. Like you're at a buffet and you just can't stop. So here's the problem I see is when you talk about this stuff, I really see two worlds. I said, problem. That's not fair, but there's two worlds. Thomas Larock (00:29:05): The first thing is, you have to know what question you want answered. So there're some executives somewhere and he goes, "I got questions. I need answers." You have to go and collect the data. You have to go and get the information to answer those questions. Denny Lee (00:29:21): Correct. Thomas Larock (00:29:22): What you're really talking about though, is something... It's almost like a deep state R&D project. I'm going to go get all the data. Maybe it's information. I don't know. I'm searching for an extra signal. I'm like SETI and I'm trying to find a signal that we don't know exists yet because I think it might have more information. However, in the meantime, while you're waiting six months for something to finish, the executives like, "I need red, green, yellow." I need to make a decision. I can't wait the six months. So I think there's really two fields here and I don't think people talk enough about the overlap because they always talk about how, I'm just going to fire up 10 more nodes and we're just going to process all this. And we're just going to keep looking for something that might have some value. Who knows? And you're still missing... I just need an actionable dashboard right now. Here's my question. What information will satisfy that question? And I need you to keep ensuring that the quality of the information you're giving me is of the same level. Thomas Larock (00:30:20): Now, if there's something new that you discover later, that's great, but for now I just need something now. Anyway, I just feel that a lot of times people head down the path that you're at, but then you are the edge case, right? You're building Yahoo cubes and all that. And I remember when all that was coming out and it's just wonderful, but you're such an edge case, but I think people want to be that edge case that you're at and they just want to get everything they can and look for that signal. And I'm not sure that that's where people really need to be. I think in a lot of cases, people can have like a little bit of a simpler life, but they should think about the stuff you're doing at Databricks and Spark and all that and think about it more as... It's more research, in my opinion. Denny Lee (00:31:04): Actually, I disagree with that statement, but not for the reasons you think. So, because I actually agree with, I'd say 80% of what you're saying. The reason why I say that is because what typically happens though, is that when the executive or the product manager or whatever recognizes they need that data or something new comes in, by the time they actually need it, and when you start processing it to finally integrate it in the dashboards and the reports and everything else, it's too late. Number one. Thomas Larock (00:31:39): Agree. Denny Lee (00:31:39): Number two, from an ad hoc perspective, more times than not, you don't even know what you know, until you start processing it. Now, saying what you just said, I do actually agree with you on the point, which is, you're not supposed to make this into a deep state research project where you're grabbing everything. I do agree with that wholeheartedly, in fact. Denny Lee (00:32:00): This has nothing to do with structure or anything else. This has to do purely has to do with... Look, you're storing all this stuff. There's actually a couple of really important things to call out. One, you're spending money to store it. If you're storing that much data, it's going to cost money. Do you need to store all this stuff? Number two, do you have privacy concerns by storing all of this data? You can't just store data and for the fun of it and not taking the [inaudible 00:32:28] that you actually have security protocols, you have privacy protocols that you actually have to care about. Sorry, you do. Okay. And this is before I get into HIPAA health act, GRC compliance of CCPA, any of that stuff, right? That's a whole other conversation. So you actually have to care about these things. You're not supposed to just go in and randomly report stuff. Denny Lee (00:32:46): So like I said, I actually agree with the sentiment in what you're talking about. What I think the only difference between... And where the 20% arrives is that when you are a moderately size or larger company, what the concern really is is that you really don't know what you don't know. And if you're going to be processing any data when you're a moderately sized company or larger, you ultimately need to process a lot more data than you want even, in order to get to that actual dashboard. Does it replace the actual dashboard? Quite the opposite. It means you need to create better ones faster. We're actually not that far apart. It's just more that part about saying, okay, ultimately we don't know what we don't know. So if you're a moderate sized company, you're going to have to probably process more data, but you have to respect the data. Thomas Larock (00:33:39): I actually think we're closer than the 80% because I did leave that part out where that data, if you're collecting the right data, it should lead you to ask more questions about the data. I do agree with that. I think my point was when people think about some of these projects, there's not enough structure around it. Like, "Hey, what's the information I need right now for what I can do. And then what's the other stuff that I have to go and look for." And yes, good data should lead to good questions. "Hey, I'm curious. Can we do this other thing too?" Now we're talking now it's going to take four weeks. Now, it's going to take six weeks and that's okay. And that's what I call that research part. But you only get there by asking the exact questions. Denny Lee (00:34:17): Exactly. You should never start with the context of like, "Let me just grab everything," because I'm like, no, no, no, no, no. This is a cost problem. This is a security problem. There's a privacy problem. There's all sorts of problems. And you don't start that way. Anybody that ever starts that way will fail. Unequivocally will fail their project. And I'm going like, "No, no, no, it's newer." I'm like, "No, same problem in the data warehousing world." The same problem. Thomas Larock (00:34:39): But that's a problem for future you [crosstalk 00:34:44] you today, you don't have to worry about that. That's a problem for future [inaudible 00:34:48]. Denny Lee (00:34:48): Yeah. I guess if you follow the idea that I'll just switch jobs every two years and then I can run away. Sure. I guess that's fine, but I would hope that all of us at least, when we're doing this podcast, we're actually trying to advise people who actually want to provide value authorized [crosstalk 00:35:03] Rob Collie (00:35:05): Given the truthiness of more data being created in the last five seconds than in all of human history, Denny's going to have to have me changing jobs more than every two years. Right. Denny's had a larger number of different employers in the last week than most people have in seven lifetimes, just to keep escaping the data storage. So let's get back to the linear progression. Right? We had started with data warehousing, turn everything into rectangles, incredibly expensive, incredibly slow, even just to get it stored. Then we went full semi-structured Hadoop, which has delayed the rectangularization, schema on read. I'm going to just drop that right in there. I'm just practicing. I'm trying it on- Denny Lee (00:35:49): We're there for you, man. Rob Collie (00:35:51): ... But it was a two week query time. So then along comes Spark and now it's a lot faster. And we were starting to turn the corner as to, we need something that resembles structured rectangular style... I don't know. I'm going to use the word storage, but I'm very tentative about that word. We need concept of structure as close to the semi-structured storage as we could possibly get. I don't think we finished that story, but I'm definitely not yet understanding is this where we turn the corner into Delta Lake and Lake House or is it Databricks? What are we- Denny Lee (00:36:28): No, no. It's actually, is the turn of [inaudible 00:36:30] Delta Lake and Databricks and Lake House for that matter, because that's exactly what happened. So at Databricks, the advantage of us using Spark and helped create it, is that we were now working with larger and larger customers that had to process larger and larger amounts of data. And they're so large that basically we have to have multiple people trying to query at the same time. And so you remember old database, the idea of like, do I do dirty reads, do I have snapshots rights, things of that nature. So what invariably happened with almost every single one of my larger customers, was that all of a sudden this idea that your job's failing, and that's normal, right? Your jobs failing, but they'll restart. But how about if they fail, fail? Denny Lee (00:37:14): What ends up happening is that these files are left over in your storage. And this is any distribute system, by the way. This isn't a specific to Spark. This is any distribute system that's doing right. If it's doing a distribute multiple tasks, a job that runs multiple task, that's writing those multiple tasks onto multiple nodes with multiple nodes or writing to disk of some type storage of any type, invariably something fails. And so, because something fails, all of a sudden you're left over with a bunch of orphaned files. Well, anybody that's trying to read it is going to say, "Wait, how do I know these files are actually applicable versus these faults actually need to be thrown away?" You need this concept called a transaction to clarify which files are actually valid in your system. So that's how it all started. It all started with us going backwards in time, recognizing the solution was already in hand, we've been doing it for decades already with database systems, we needed to bring transactions into our Data Lake storage. Rob Collie (00:38:17): Quick, an important historical question and both of your histories, Denny and Tom, have you had experience? Have you performed dirty reads and if so, were they done dirt cheap? All right. Had to do that. Thomas Larock (00:38:34): So in my answer, my answer, Rob is yes. I was young and I needed the work. Rob Collie (00:38:42): I mean, now we need to write the whole song. Right? Thomas Larock (00:38:45): I'm just going to tweet it. [crosstalk 00:38:48] Tweet it so I can [crosstalk 00:38:50]. Denny Lee (00:38:50): At this point we literally could do a trio here without any problems because we know for a fact you qualify. Rob Collie (00:38:55): So, is that ultimately like tagline for Databricks, dirty reads done dirt cheap, but it's not dirty because it's transactional. Denny Lee (00:39:03): Exactly. Rob Collie (00:39:04): I think the world would really respond. Well, the problem is, is that we're now old enough that that song is like the Beatles. Denny Lee (00:39:11): Yeah. Yeah. That's true. We should probably provide context to our audience here. Thomas Larock (00:39:18): Wow. Rob Collie (00:39:19): That is an old AC/DC song. Dirty Deeds Done Dirt Cheap. That's the album title too. Thomas Larock (00:39:23): I think all four Beatles were still alive when that stuff- Denny Lee (00:39:26): Yes, all four Beatles were alive during that song. Rob Collie (00:39:29): John Bonham from Zeppelin might've still been alive. Thomas Larock (00:39:35): So, we're aging ourselves to our entire audience. Thank you very much. Rob Collie (00:39:39): All right. All right. All right. And dad joking to the extreme. Thomas Larock (00:39:42): We've thrown around the term, JSON a lot. Rob Collie (00:39:44): Can we demystify that for the audience? I actually do know what this is but like- Thomas Larock (00:39:49): It's call hipster, XML. Rob Collie (00:39:51): JSON equals hipster XML? Thomas Larock (00:39:53): Yes. Rob Collie (00:39:53): Okay. All right. This sets up another topic later. Denny, would you agree that JSON is hipster XML? Denny Lee (00:39:59): I absolutely would not, even though I love the characterization, but the reason why is because I, [crosstalk 00:40:06] Hey, Rob. Yeah. Yeah. I'm saying it. Rob Collie (00:40:12): Me too. Okay. Thomas Larock (00:40:14): See. Is Jason a subset of XML? Denny Lee (00:40:15): JSON is an efficient way for semi-structured data to actually seem structured when you transmit it. Rob Collie (00:40:25): Okay. So, it's the new CSV, but with spiraling, nesting, curly structures. Denny Lee (00:40:30): Correct because it allows you to put vectors in a raise quite efficiently and allows you to put [Nyssa 00:40:35] structures in efficient. Rob Collie (00:40:36): So it's a text-based data format, right? Denny Lee (00:40:38): Correct. Rob Collie (00:40:38): ... so that it's multi-platform readable- Denny Lee (00:40:41): Exactly. Thomas Larock (00:40:42): Yeah. It's XML. Denny Lee (00:40:44): No. Rob Collie (00:40:45): It's hips and mouth. Denny Lee (00:40:46): So, I believe I have war stories probably because of you Rob about all... Especially when reviewing the XML A. Rob Collie (00:40:57): Oh yeah. Well, listen, I don't have a whole lot to do with that. Denny Lee (00:41:02): [crosstalk 00:41:02] I'm just saying, Tom, you will vouch for me. The insanity of XML, right? You will vouch that. Yes, please. Thank you. I'm supposed to figure out the queue structure with this thing? My VS, visual studio is collapsing on me right now. Rob Collie (00:41:21): Well, hey look that XML A stuff which had nothing to do with creating, is the thing that we talked about with Brian Jones on a recent episode. That's the stuff that was saved in the Power Pivot file as the backup so that we could manually edit that and then deliberately corrupt the item one.data file in the Power Pivot stream and force a bulk update to like formulas and stuff. And yes, it was a pain in the ass. Okay. So JSON, it's advanced CSV, it's XML like, it's the triple IPA of XML or is it the milk stout? Is it the milk stout of XML? Denny Lee (00:41:58): I'm not even partaking in this particular conversation? I'm just- Thomas Larock (00:42:02): Honestly, JSON would be like the west coast hazy IPA. Okay- Denny Lee (00:42:07): Okay. Fine. I will agree to that one. You're right. You're right. West coast hazy IPA. Fair. That's fair. Rob Collie (00:42:12): All right. All right. Okay. Well, I'm glad we I'm glad we did that. I'm glad we went there. And the majority of... I'm going to test this statement... The majority of Data Lake storage is in JSON format? Denny Lee (00:42:23): No. Actually the majority is in the parquet format or ORC format, by the way, depending on it. But which is basically for all intents and purposes a binary representation from JSON into a column store. Rob Collie (00:42:34): I'm just going to pretend I didn't ask that question. I'm going to move on. All right. So is it the notion that when you're reading from Spark, which is of course reading from other places that because things are being updated in real time, it has unreliable reads. Denny Lee (00:42:52): So it's not just Spark. Any system. Rob Collie (00:42:55): Sure. Why did Spark need Databricks? Denny Lee (00:42:58): Fair enough. And I mean, it's more like in this case, honestly, it's why does Spark need Delta Lake? And to be fair to the other systems are out there. And like I said, there's iceberg Hadoop as well, but I'm going to just call out Delta Lake. Right. But the reason was because it's very obvious when you talk about streaming. If I've got two streams that are trying to write to the same table at the same time, and one invariably wants to do an update while another wants to do a deletion, that's a classic database transactional problem. Rob Collie (00:43:25): Even like a Dropbox problem, right? You have multiple users in Dropbox. I get merge conflicts all the time. Denny Lee (00:43:30): Right. So that's exactly the point, right? What it boils down to is that when you get large enough in scale, I don't mean size of your company. I just mean the amount of data you're processing in which you could conceivably have multiple people trying to write or read or both at the same time, you could solve that by two people trying to do the same thing at the same time, and you'd still have the same problem. Why did databases become awkward? That was one of the key facets that we could either succeed or fail. It was very binary. It's succeeded or failed. So we knew whatever went in, it got in, or if it failed, you're given an alert and you're told, "Guess what? It didn't work. You need to try again." Right. Same concept. That's what basically it boils down to. It's like, if you're going to be using your Data Lake as a mechanism to store all of your data, will then do not want transactions to protect that data so that it's clear as daylight what's valid and what's invalid. Denny Lee (00:44:31): So I'm not even trying to do a product pitch at this point. I can do that later, but I'm just saying... It's like, no, just start with that statement. When you have a Data Lake, you want to basically make sure whatever systems processing it, whatever systems reading it. I don't care which one you're using honestly. Obviously I hope you're using Spark, but the fact is I don't care. You use any system at all that's trying to read or write from it. Don't do you not want to make sure that there are some form of consistency, transactional guarantees to ensure what's in there is valid. And then once you've accepted that problem, that this is a problem that you want solved, then invariably that'll lead you to the various different technologies. Denny Lee (00:45:09): Again, I'll be talking about Spark and Delta Lake because I think they're awesome, but the reality is like, that's why the problem, right? That's why we realized this was crucial for most people. Incidentally, that's why we open sourced Delta Lake because we're going like, "No, it's so important that I don't care which platform you're using it on." I don't care if you're using it on Databricks. I don't care if you're using Spark. We just don't care. Denny Lee (00:45:34): Because the whole point is that you got to trust your data first. And if you can't trust your data, forget about machine learning. Forget about BI. Forget about all of these other systems that you want to do downstream. I need to make sure whatever store my Data Lake is actually valid. And then a lot of other people will tell me like, "Oh yeah, well maybe if you could just shove into a database or chuck it over, all this other stuff, I'm like, don't get me wrong. I'm all for databases. Just because I'm a smart guy, doesn't mean I hate databases. Quite the opposite. I've run my own personal Postgres and my SQL still to this day and SQL server. Yes. I have a Window box somewhere in this house so I can- Thomas Larock (00:46:05): See if it runs on Linux. Denny Lee (00:46:08): SQL can run [crosstalk 00:46:11] No, it's true. It's true. But I'm still old school. I actually, no joke, still have a running at least I should say I used to still have a running 2008 R2 instance somewhere. The Thomas Larock (00:46:19): The Lee household, by the way, doesn't actually ever need heat. [crosstalk 00:46:23] It's heat by CPU. Denny Lee (00:46:25): Right. All the stick at CPs and GPS. I can actually cook an egg now. Yeah. Rob Collie (00:46:33): It's like the most expensive power way to cook something. Denny Lee (00:46:35): Exactly. Yeah. Thomas Larock (00:46:37): So he doesn't touch a thermostat. He just spins up like a hundred nodes. There you go. Denny Lee (00:46:41): Yeah, yeah. Rob Collie (00:46:42): That recent heat wave in Seattle was actually when Denny's air conditioning failed and it started pumping all that heat out into the atmosphere. Denny Lee (00:46:50): Yeah. Oops. Sorry about that guys. My bad. Rob Collie (00:46:52): Is it El Nino? No, it's Denny. Denny Lee (00:46:55): Thank you. I'm already in enough trouble as it is Rob. Do you really need to add to the list of things? I mean, I'm in trouble for now. Rob Collie (00:47:02): We're going to blame you for everything. Denny Lee (00:47:03): Oh, fair enough. No, that's all right. That's completely fair. But the concept's basically, it's like, because of its volume, it's sitting in the Data Lake anyways. Do I want to take all of it and move it around or somewhere else? And I'm like, I'm telling you. No, you don't. That's the exactly the Yahoo promo. I know it's sort of funny, but I'm really going right back to Yahoo. When we had this two petabyte system, we were moving hundreds of terabytes in order to get in to that cube. I'm going, "Why, why would I want to do that?" And especially considering we had to basically update it with new dimensions and new everything like every month or so, which meant that we were changing the cube, which meant we're changing the staging databases that was in... Which was basically this massive Oracle rack server and then against your petabytes of data. The whole premise is like, I obviously want dashboards, but I don't want to move that much data to create the dashboards. Rob Collie (00:47:59): Sure. My jaw is on the floor at this point and oh my God, we didn't have the concept of transactions in Data Lakes from the beginning? Denny Lee (00:48:11): That's correct. Rob Collie (00:48:12): Oh my God. Thomas Larock (00:48:14): It's just a lake. Denny Lee (00:48:14): Yeah, it's a lake. [crosstalk 00:48:16]. Why would you bother? All right. Thomas Larock (00:48:19): So the idea, because- Rob Collie (00:48:20): ... multiple people are peeing in that lake at the same time. Denny Lee (00:48:23): Well, there you go. So people were under the perception that people weren't peeing in the lake, so the lake is perfectly fine. And then in reality, not only are people peeing in the lake, you got all sorts of other duties inside there. So yes, I'm using duty. Rob Collie (00:48:39): You've got streams crossing, you've got all kinds of things. So I'm absolutely getting smarter right now. And I'm super, super, super grateful for it. Where do I like to get smarter? That's right in front of an audience. That's the best place to learn. Everyone could go, "Oh my God, you didn't know that." Denny Lee (00:48:54): I think Tom can vouch for me though. The fact that you're getting smarter with me on is not a good testament for you, buddy. Thomas Larock (00:48:59): No. Rob Collie (00:49:00): Ah, no. Come on. You're something else, bud. So Denny Lee (00:49:02): That's right. Something else, but that doesn't mean smarter. Rob Collie (00:49:07): I think your ability to bring things down is next level. Okay. So transactions. I'd heard about Databricks for a while, but in prepping for this, I went and looked at your LinkedIn. Denny Lee (00:49:20): Oh wow. Rob Collie (00:49:20): ... and I saw these other terms, Delta Lake and Lake House, but Databricks has existed longer than those things. Is that true? Denny Lee (00:49:29): That is absolutely true. Rob Collie (00:49:29): And the company is called Databricks. Denny Lee (00:49:31): Correct. Rob Collie (00:49:32): So what is the reason for Databricks to exist? Is it because of this transactional problem or is it something else? Denny Lee (00:49:40): No, actually that's where I can introduce the Lake House. So if I go all marketing fun just for a second, like you use your data bricks to build your lake house. Bam. Rob Collie (00:49:51): Oh no, no. Denny Lee (00:49:55): Yeah. I did that. I did. No, no. Data bricks are what you use to build a data factory and a data warehouse. Now you live in the Data Lake house, right? No. You make the data bricks to build your Data Lake house. Absolutely. I don't need a data factory. I'm building a beautiful Data Lake house. It's right on the water. It's gorgeous. I'm sitting back. I'm listening. Yeah, no- Thomas Larock (00:50:15): Again, no. The Data Lake house is part of your data estate. I get that. Okay. But to me, you're using the Databricks for the data warehouse, the data factory. And do you shop at the data Mart? I get it. Rob Collie (00:50:27): I'm trying to be so deliberate here. So look, we're trying to follow a chronology. We want a number line here. And so first there were Databricks. Why Databrick? Denny Lee (00:50:39): Why Databricks is because we wanted to be able to put some value add initially on top of Apache Spark. Right? So the idea that you can run a Apache Spark with it's open source is great, and lots of companies have been able to either use it themselves without us. Or there are said cloud providers and vendors that are able to take the same open source technology and build services around that and have benefited greatly for doing that. Databricks decided to not go down the traditional vendor route because the decider say, we're going into the cloud right from the get-go. At the time that we did it, it seemed really risky. In hindsight, it makes a lot of sense. But at the time that we did it back in 2013, seemed really risky because they're going like, "We could get lots of money for on-prem customers," because that's where the vast majority of customers work. Denny Lee (00:51:30): But where we saw the value was this concept that with the elasticity of the cloud, there is going to be a whole different set of value add that Spark and the cloud together will bring that you can't get on-prem. The idea that I can automatically spin up nodes when you need them, if you ever log into Databricks, the idea is like we default between two and eight nodes. So you start with two worker nodes and then basically you'll scale up to eight automatically. But just as important scaled out, just as important. So the idea that once you're done, we're not leaving those eight nodes on. If we're seeing no activity, [inaudible 00:52:08] those nodes go back down. You're not running. It's idle after 60 minutes. That's our default. Fine. We're just shutting it down. The idea that instead of you writing lots of code yourself, you've got a nodebook environment. Denny Lee (00:52:22): Not only does it make it easier to write it, but also to run your queries, but also has permissions that also has commenting. But also on top of that, if you're closest to shut down, everything's still safe. You can still see the nodebook. You can see the output of the nodebook still saved for you, but you're not paying for the price of a cluster. Right? So this is what I mean by value added. So that's how we started. Right. And so that's how plenty of people who were into machine learning or to just big data processing. They got interested in us because we're the value add was that now they didn't have to maintain to configure all these Spark clusters. It automatically happened for them. They were automatically optimized right away. Rob Collie (00:53:05): So it's probably the wrong word, we'll get there by depth charging around it a little bit. So was Databricks when it first came out, was it a cloud-based like layer of abstraction and management? Denny Lee (00:53:17): Yeah. Rob Collie (00:53:17): ... across Spark nodes? Denny Lee (00:53:18): Exactly. That's it. Rob Collie (00:53:21): I I hit the sub with the first depth charge. Denny Lee (00:53:23): And all sources were assessed or passed, however you want to phrase it. Rob Collie (00:53:26): All right. So that's Databricks. Denny Lee (00:53:27): Yeah. And so just to give the context, every single technology that we're involved with, whether it's the advancements of Apache Spark to go from, for example, you had to write the stuff initially in Scala, which is how- Rob Collie (00:53:42): [inaudible 00:53:42]. Denny Lee (00:53:42): So credit to Scala... but then you had to write it in Python, right? But then over time we added data frames with the [Smart 2X 00:53:50] line, all of a sudden, now this concept of actually running in SQL, because that makes a lot more sense for everybody. And the Smart 3.0, which includes ability for the Python developers to interact with it better. Or that when we introduced Delta Lake or when we introduced MLflow, all of these different technologies were the realization of as we're working with more and more customers, what were some of the parts that really are needed for the whole community to thrive, irrelevant of whether they're on Databricks or not and which parts are going to be the parts where we will quote unquote, keep for ourselves because we're the value add. Denny Lee (00:54:28): We're going to provide you something valuable on top of said open source technologies, so that way you can still benefit from the learnings. So a [inaudible 00:54:38] and MLflow and Smart, for example, using those technologies, you can still benefit from everything we're saying. It's like, we're still publishing reams of white papers and blogs telling people how to do stuff, because that's the whole point. It's basically a large educational push. We want everybody to grok that there's a lot of value here and here's how to get that value. And then Databricks, if we can say, yeah, but we can make it faster, we'll make it simpler or make it whatever, that's where we will be valuable to you. Now, bring it back to the Databricks to the Lake House concept, if you think about purely from a, why did we use the term Lake House, what it boils down to is the whole value of a data warehouse was the fact that you could protect the data, you had asset transactions. Denny Lee (00:55:21): You could store the data, you could trust what was being stored and you could generate marts off of it to do your business dashboards, whatever else. That's the whole premise, this one central repository. Okay. So where Databricks came in, it was like, well, in a lot of ways, we gave away a cyclotron in the house. We gave away Spark for the processing. We gave away Delta Lake for the transactional protections. On the machine learning side, we're even giving away [inaudible 00:55:46] But the things that there's also all these other technologies like TensorFlow for deep learning, your Pandas, your scikit-learn for machine learning, all these other things, right? Denny Lee (00:55:53): There's all these other frameworks put together. So the premise is that as opposed to, we grew up where it was relatively unfragmented by the time that we got into database systems and SQL server and things of that nature. Right now the system is still massively fragmented with all these different technologies and it'll stay that way for a while not because there's anything wrong, but because we're constantly making advancements. So the value add, what we do is basically saying, "Okay, well, can you make everything I just said, simpler?" Number one. And then for example, now back to the in-memory column store, when you look at Databricks and we said, we have to make Spark faster. So we made Spark as fast as we possibly can. But the problem is that when you're running Spark in general, there's the spin-up time to build up your tasks, spin up time to run the jobs, spin up time to do the task. Denny Lee (00:56:47): There's an overhead. Now that overhead makes a ton of sense for what it's use cases for, which is, in this case, I have a large amount of data. I need to figure out how to process that. But what's a typical BI style? Of course, you already have it structured. You already know more or less what you have to work with. It's just go do it. We can push Spark to a point, but we can't get any faster because at some point literally the JVM becomes a blocker and the fact that we have this flexibility to spin up tasks to analyze the data becomes the blocker. We're not about to remove the flexibility of Spark. That seems silly. So what does Databricks do? And this is one of the many features of quote unquote, the Lake House that Databricks offers, which is, okay, we built a C++ vectorized column store engine. Denny Lee (00:57:36): So how can you do BI crews on your Data Lake? Guess what? We've written in C++. We're going back old school back to our day in order to be able to work around that. We can make some general assumptions about what BI queries are. So since we can make those assumptions, the aggregate step you're making, even distincts though, that's always a hard problem anyways, right? The joints that you're making, the group [eyes 00:57:58] you're making, right? You can make these types of assumptions. If you know the structure of the data, like in this case, because even with Delta, what's Delta? Underlies Parquet. Well, Parquet in the footer has a bunch of statistics. So that tells us basically, what's your min-max range. What is the data types you're working with? So you can allocate memory right from the get-go in terms of how much space you need to take up in order to be able to generate your column store, to do your summaries, to do your subs, your ads, or anything else. Denny Lee (00:58:24): So since you have all that in place, we can simply say, "Let's build a column store vectorized engine in C++ that understands the Spark SQL syntax. So the idea that you haven't changed your Spark at all. If the query you're running because of taking a UTF, because it's hitting whatever, doesn't get the photon engine. It's okay. We'll default right back to Spark and you're good to go and Sparks pretty fast. But if we can use the photon engine, bam, we're going to go ahead and be able to hit that and we can get the results back to you significantly fast. And so for us, this is an example of what I mean by us giving you value add. And the fact is, over time the value adds are going to change. And that's actually what we want. We want an advancement of the technology and we're taking the bet that we will always be able to go ahead and advance the technology further, to make it beneficial for everybody. Then it's worthwhile for you to pay us. Rob Collie (00:59:18): Photon, koala, Panda, Cloud [crosstalk 00:59:25] . You know what, Tom? Thomas Larock (00:59:26): Yeah. Rob Collie (00:59:27): More data platform technologies were invented in the last year than in all of human history. Thomas Larock (00:59:34): That might be true. [crosstalk 00:59:37]. Rob Collie (00:59:36): The real rate of expansion here is the number of technologies. We go decades where we have SQL and then someone comes up with OLAP and then like things like ETL come along. It's like four or five total things over the course of decades. Thomas Larock (00:59:55): They just get renamed. Rob Collie (00:59:56): Yeah. Yeah. A lot of the same problems keep rearing their head, but in a new context. Denny Lee (01:00:01): And exactly to your point, right. Asset transactions came back and rightly so. It rightly so came back. And like I said, what did we build photon on? We're pulling the old [inaudible 01:00:14] Mary Collins store stuff that we did, dude. We're pulling back to that day. Rob Collie (01:00:18): So Photon is that C++ written thing. Denny Lee (01:00:22): Yeah. Rob Collie (01:00:23): Okay. And that is similar to, in a lot of ways like the VertiPaq engine that underlies power- Denny Lee (01:00:30): Yes. Very similar. It's in memory called start engine, dude. Thomas Larock (01:00:35): So I got to drop here in a couple minutes, but I want to just say the benefit of us being old, I mean experienced, is that we recognize all this stuff has already happened. So I read this article a couple of weeks ago. This guy, it was a dev ops article and he's like, "We need a new dev ops database." And I look at that. I go, "What are you talking about? You need a new dev ops database?" And he goes, "Do you know how hard it is to merge changes when you're both trying to update the same database and you have to roll this thing back?" And I'm like, "Dude, this is a problem for 40 years." It's not that you need something new. It's like, you just need a better process for how you're doing your data ops. Your data ops process is failing you and it's not because of the database part of it. It's because of how you've implemented your pipelines and things. Thomas Larock (01:01:27): And I just sat there shaking my head and I go, the kid that wrote this, he's like 22 years old and he's never had this issue like with Denny has. What if you want to update and delete in this Spark group of clusters. He's never had to fight through that yet. So to him, it's all new. Right? And he's like, "Oh yeah, we totally need a new thing." And now he's going to go reinvent hipster, JSON and he's going to say, "Now I've solved this." And bam, now you've got a new standard. Right. And this is why we have so many different data technologies and names. Rob Collie (01:01:57): Just alienated every 22 year old techie on the planet. Do you know how hard it's going to be to get someone to buy you a hazy IPA now? Denny Lee (01:02:06): Yeah, I do. Thomas Larock (01:02:08): I'm okay with that. I'm in the scotch phase right now. Denny Lee (01:02:10): So fair enough. Fair enough. Highland park, by the way. Thomas Larock (01:02:14): Yeah. I'm good. I'm good with all that. So if you're 22 and listening to this, first of all, I'd say that's just not possible. Rob Collie (01:02:24): Yeah. You're probably not, but hey, if you are a 22 year old listening to us, let us know. Denny Lee (01:02:29): Yes, please. Rob Collie (01:02:31): Tom wants to buy you a scotch. Denny Lee (01:02:33): Exactly. There you go. But also I do want to emphasize the fact that for folks that are old-school data based types, the fact is that you're listening to three of us. World school database [inaudible 01:02:48] and a lot of the, maybe not the technology itself, but a lot of the concepts, a lot of the processes are very much applicable today as they were 20 years ago. Just because you're older does not mean you can't keep up with this technology. You just have to recognize where the old processes actually are still very, very, very much in play today. Thomas Larock (01:03:15): I can vouch for that as I've been trying to venture more into data science. My experience as a data professional and my math background as well, has blended together to make it an easy transition where... And I'm looking, I go, this ain't new. Denny Lee (01:03:29): Exactly. Thomas Larock (01:03:30): Kind of the same thing. Rob Collie (01:03:31): Let's change gears for a moment because I'm getting closer to understanding what you're up to. All of this Linux world stuff, that's the world you run in these days. Denny Lee (01:03:42): Yeah, that's what they tell me at least. Rob Collie (01:03:45): For a lot of people listening, I suspect that this sounds like a completely alternate universe. Denny Lee (01:03:52): Fair enough. Rob Collie (01:03:52): A lot of people listening to this are one form or another like Power BI professionals and very, very much up to their eyeballs in the Microsoft stack, which I know isn't mutually exclusive with what you all are working on. That's one of the beauties of this brave new world. However, a lot of people don't have any experience with this even though they're doing very, very, very sophisticated work in data modeling, DAX, M. They're hardcore professionals. And yet a lot of this stuff seems like, again, like a foreign land. So where are the places where these two worlds can combine? What would be the relevance or like the earliest wave of relevance. What's the killer app for the Power BI crowd? Denny Lee (01:04:38): Yeah, so the Power BI crowd, what it boils down to is, whatever's your source of data. If you're talking about a traditional, like hitting a SQL server database, yeah, you can... Well, 99.9% chance you can trust it, right? There was transactional protection. The data wasn't there. You're not getting dirty reads unless you, for some reason, want them you're good to go. But what ends up happening to any Power BI user, is that you're not just asked to query data against your database anymore. You're asked to query against other stores and you're asked to query against your Data Lake. The Data Lake is the one that contains the vast majority of your data. There's no maybes here. It is the one that contains the most of your data. So the problem underlying which isn't obvious, and I'll give you just a specific example to provide context and I'll simplify. Denny Lee (01:05:30): Let's just pretend you've got a hundred rows of data. And then somebody decides to run an update statement. Now, in the case before Delta, how you run an update statement, let's just say the update affects, let's just say 20 many rows. You actually have to grab all 100 rows, rewrite them down, rewrite 80 of them. Take the 20 rows that you need to update, rewrite them. They're into a new table. Once you validate that it works, delete the original table, rename the new table back to the old table. And now you have the correct number of rows you want with the 20 rows as an update. So far so good. What happens if you're trying to query that at the exact same time when it's mid-flight? Thomas Larock (01:06:21): What happens? Denny Lee (01:06:22): Exactly or what happens if you are trying to go ahead and create when multiple users are trying to do the same thing, because for sake of argument, two people are trying to run the same 20 row update at the exact same time and because it's in` mid-flight, neither one knows which one is the primary. So that means the data you get, you can't trust if there's anything that's done to it. Maybe a deletion happened. And also now there's only 80 rows. Right? And they're going, "Okay, so which one is it?" Or the worst scenario. You take the hundred that you had, it fails, mid-flight. It wrote the 80 rows down into the same folder. So what you end up having when Power BI is trying to query it, it's not getting a hundred rows. First of all, it's getting a hundred of the old rows, it's getting 180. So now your numbers are completely off. And so that's the context that when you had a database the transactions, you didn't have to worry about that. So what's the value of the Data Lake, having Delta Lake? Denny Lee (01:07:32): That's exactly it. Having that ability to protect the data. So even if it was mid-flight writing and it failed, it doesn't matter. There's a transaction log that states very clearly, "Here are the 100 rows that you care about. And it's really the files by the way, that's in the transaction log. But let's just for sake of argument, here's the five files that have the 100 rows you care about and that's it. The only reason I'm calling that stuff out is because this is also very important for clout. So when you go ahead, whether it's Windows write DIR or Lytics you write LS, right, that's a pretty fast operation. When you run that same command, LS on a cloud object store, it's actually not a command line operation that you're used to. What it is, is actually translation to a bunch of REST API calls. And so the REST API calls basically underneath the covers is basically a distributed set of threads that go out to the different parts of the storage and return that information back to you. Denny Lee (01:08:34): Now for five files probably it doesn't really matter, but if you're talking about hundreds or thousands of files, just listing the files takes time. So just running the LS, isn't going to come back in seconds. It will take many seconds to minutes just to get that query back. So how Delta Lakes solves that problem, it says, okay, wait, no, it's okay. In the transaction log, here are the five or 100 files that you need. So there's never a listing. So whether it's Spark or any other system that's querying Delta Lake, the transaction logs telling you, "No, here's the five/100 whatever number of files that you actually need. Go grab them directly." Please don't forget. A cloud object store itself is not a traditional obstacle. This idea of bucketing this idea of folders. The folders don't actually exist. It's just something that you have to parse. It's just one gigantic blob of crap. That's all it is. So what happens is they actually have to basically parse the name of the files in order to return that to you and then claim there's a folder in there. Rob Collie (01:09:36): It's kind of like schema on read, right? We're going to give you this notion of directories, but it's created from thin air. Denny Lee (01:09:41): Exactly. It literally is. Yeah. So because of that, then the whole premise of saying, "Okay, well now I can return that stuff to you that much quicker." And then there're other aspects of Delta Lake, for example, like schema evolution and schema enforcement. The idea that if you've already declared that this is the schema, like as in, I've got two wins in a string column, let's just say. If you try to insert, update into that table, and it's not two string, maybe it's two [inaudible 01:10:09] strings, let's just say, it'll say, "No, I'm not going to let you do it because I'm enforcing the schema," just like a traditional database staple would. But also we allow for schema evolution, which is, if you then specify, no, no. In this case, allow for evolution, go ahead and do it. Right. So it gives you the flexibility while at the same time giving that structure. Rob Collie (01:10:32): It's almost like even... There's a really simple parallel here, which is like an Excel validation. You can say, "Here's the list of things you can choose from," but then there's also allow people to write in new values or no? Denny Lee (01:10:44): Right. Rob Collie (01:10:46): So there's that nature of flexibility. It's either hard enforce schema or evolvable. Denny Lee (01:10:52): Exactly. No. And that's exactly right. So there are many factors like that. I can go on about streaming and batch and all these other things, but the key aspect, what it boils down to, for any Power BI user, any Power BI professional is this notion that the Data Lake, without asset transactions, without things like schema force, but without inherently is an untrustworthy system for various reasons. Rob Collie (01:11:19): Totally. Yeah. I mean, again, this is the jaw dropping thing for me. It's like really? That's been okay ever? Even for five minutes, that was okay? It's really hard to imagine. Denny Lee (01:11:29): Well, I mean, the context, don't forget is because you're at the tail end of what has happened to that data. The people that made this decision were on the other end, which is, I have so much data coming into me at such a disgustingly fast rate. I just need some way to get it down. Otherwise, I will lose it. Rob Collie (01:11:54): Yeah. It's a real immediate problem. And earlier you very graciously grouped me in with yourself and Tom, when you said, we're all old database people, but I was never really a database person. I've always been a lot closer to the faucet than the plumbing. Denny Lee (01:12:10): No, fair. Rob Collie (01:12:11): And so for the Power BI crew that's listening, which is again, closer to the faucet, we could say, "Hey, this is not our problem. It's not actually something that most Power BI people are going to be dealing with." It's going to be an infrastructural decision made. It is very much an IT style decision as opposed to this new hybrid model of what we used to call self-service. But it's really like hybrid model of Agile business driven faucets. But if my IT organization decides that the Data Lakes that I've been using to power some of my beautiful Power BI, it might be that I've been quietly... And this is the scary part, unknowingly suffering the consequences of crunchy rights that are conflicting with one another. Rob Collie (01:13:01): I might've been dealing with bad data and not known it, but if my IT organization decides to roll out something like Delta Lakes, do I notice? I mean, other than the fact that I won't have bad data anymore, will I need to do anything differently? Denny Lee (01:13:17): No. Rob Collie (01:13:18): Do I need to query it differently through- Denny Lee (01:13:20): Nope. Nope. Rob Collie (01:13:21): Or is it just the people who are doing the rights that have to play ball? Denny Lee (01:13:25): The way I would phrase it is this way. It's the traditional Power BI reporting problem. Why you have to care, which is the problem isn't so much that you're supposed to tell infrastructure what to do. The problem is you're going to get blamed when the numbers are wrong. Rob Collie (01:13:42): Sure. Denny Lee (01:13:43): Right. And you're the first line of people that will be attacked. Rob Collie (01:13:51): [crosstalk 01:13:51] comes out of the faucet. Right? Denny Lee (01:13:53): Yep. Rob Collie (01:13:53): I give cups of that to the rest of the team. They're going to say, "Hey, you gave me bad water." Denny Lee (01:14:01): That's right. Rob Collie (01:14:02): And I'm not going to be able to talk about the plumbing that they don't see because it's all behind the wall. It's just the faucet. Denny Lee (01:14:08): Exactly. But at the same time, exactly to your point. When you're running that query, for example, using Spark or for that matter, anything that table will talk to Delta Lake, no. Nothing changed. The only thing that changed, which is a benefit and not a con, is if you want to go back and look at historical data, Delta Lake includes time travel as well. So- Rob Collie (01:14:34): Snapshots. Denny Lee (01:14:34): Yeah. Snapshots of the previous time. So if you want to go, you can just append, like for example, you run a smart SQL statement, which is very close to SQL tables. Select column A, B whatever from table A. So then you can basically that's what your normal Power BI source query would be. Well now, just for sake, however you want to look at the snapshots, select star column from table A version, as of whatever version you want to look at. Rob Collie (01:15:01): This is another old familiar problem. So for example, our Salesforce instance, it does have some history to it, but like it's not... Or let's take an even easier example, like QuickBooks. QuickBooks is almost inherently a system that's about what's true right now and to do any trending analysis against your QuickBooks data, it's hard, right? You've got to be doing snapshots somehow. And so to run our business, we have multiple line of business systems that are crucial to our business and we're pushing snapshots into, most of the time, I think Azure SQL in order for us to be able to keep track of where we're trending and all that kind of stuff. So you're talking about that Delta Lake Lake House, give me this, snapshotting against my stores. And I don't think it's just Sparks stores. Right? It's all kinds of stuff, isn't it? Denny Lee (01:15:59): Spark is the processing engine. Right? Sort of the query agent. Delta Lake is the storage layer, basically the storage layer on top of typically Parquet. So that's the context. So the idea is that you're basically reading the Parquet file. We have other copies of the Parquet files that allow you to basically go through the snapshot. The transaction to log tells you which files correspond to which version of the data you have. Rob Collie (01:16:23): So the snapshotting, the time travel thing, right, that's a benefit that I could gain and really use as a Power BI. Yeah. That would be a noticeable difference, right? Have you kept up with Power BI very much at all. I'm wondering if in your world, if I'm using Power BI and a lot of the data that I need is stored in a Denny style world rather than SQL- Denny Lee (01:16:49): And the SQL [inaudible 01:16:50]. Rob Collie (01:16:50): Is your expectation that the import and cache mode of Power BI is still very much relevant in your world or would it only be considered cool if I was using direct query? Denny Lee (01:17:01): Oh no, no, no, no. Whether I'm using direct query, whether I'm using import, it's always going to be a product of what is your business need. For example, if SQL server suffices for everything you're doing because of the size, because whatever else, I'm the last person to tell you to go do something else. Wait, come on. We're SQL server guys. Right? So no, I'm not going to do that. That's ridiculous. Right. What I'm talking about is very much into, no, you have a Data Lake or you need one. How to get the maximum out of it. That's literally where my conversation is. In other words, for sake of argument, IT had structured it such that the results of the Data Lake go into your SQL server and then you can query your SQL server to your heart's content. Cool. I'm not saying you're supposed to go to Delta Lake directly. Denny Lee (01:17:48): I'm saying, whatever makes the most sense, because for example, I'm making this up scenario up obviously, but direct query would make sense, for example, if I have constant streaming data. I want to see at that point in time, what the change was from even a second ago or even a minute ago. Okay. Well, Delta Lake has transactional productions such that when the data is written at that point in time when you execute that... I'm using Spark SQL, as example, as the Spark SQL statement, we know from the transaction log what files have in fact been written. We will grab the files as at that point in time. So even if they're half-written files in there, it's not going to get included because it wasn't written. So then direct query for your Power BI to go grab that data. No problem at all. By the same token you turn off. I was like, "Yeah, but I don't need streaming. I just need to go ahead and augment my existing SQL table with another fact table or with a dimension table." Cool. Hit that. Rob Collie (01:18:43): Am I going to benefit from the photon engine if I'm using Power BI? Would my direct queries run faster? Denny Lee (01:18:50): Absolutely. Rob Collie (01:18:50): ... as a result? Denny Lee (01:18:50): And that's exactly the context, at least from a Databricks perspective, that's the whole point. You can take your Power BI queries and you can go ahead and run them directly, get your Data Lake with the same Spark SQL statements that you were originally running. Except now they're faster because they're using the photon engine. Rob Collie (01:19:06): Awesome. Even if I'm in import mode in Power BI, like the data refresh could also- Denny Lee (01:19:11): Exactly [crosstalk 01:19:12] would be significantly faster because now I can get the data to you significantly faster than before. Rob Collie (01:19:17): I think this has been an amazing tour of this. I would love to have you come back. Maybe we do a series, which is Denny explains it all, right? And when I say it all, I mean the domain of the Linux cool kids, Denny Lee (01:19:33): I thought we were going to talk about coffee. Rob Collie (01:19:37): Well, so I was actually... That's written down next, is espresso. Denny Lee (01:19:41): Good. Rob Collie (01:19:41): I wanted to get into that. So there's a scene in Breaking Bad where Walter White meets Gil and Gil has been like this master chemist. Gil has been working on the perfect coffee. And Walter is really obsessed about really getting into this giant lab and building and making his blue perfect mix at an industrial scale. He couldn't be more excited and yet he stops and goes, "Oh my God, this coffee, why are we making meth?" Denny Lee (01:20:12): Exactly. Yeah. Yes. Thomas Larock (01:20:15): So, yeah. I agree with you wholeheartedly. Rob Collie (01:20:18): You seem like the Walter White that decides, "Nah, you know what, it's going to be [crosstalk 01:20:24] because I watch even your latte art game. I've watched it evolve over the years. You were in like when Casper moved to Redmond, I remember him like touching base with you and getting the official espresso, like [Kit 01:20:39] so, here I am in Indiana, I'm years behind you in the espresso game. And so we just splurged for one of the automated, like espresso and latte makers from DeLonghi or whatever. And every time I tell that thing to make me a latte and it ends up like this white foam with a vampire bite where the two spouts of espresso came into it. Every time I do that, I think about you and these works of art that are crafted with... Like you got to use the word like artisanal and handcrafted and I'm pushing a button and I'm getting this monstrosity. I just go, "Maybe Denny 15 years ago would have been okay with us. But Danny of today would be very, very, very upset." Denny Lee (01:21:22): That's true. I mean, I am from Seattle. So you have to admit many of us here are very OCD. So that's why I fit in very well, for starters and saying, well, you do know this is like, again, for those of you who may not know, Seattle is a very much a coffee town to put it rather lightly. Rob Collie (01:21:42): Anytime you have a people that live under a one mile thick cloud blanket, nine months out of the year... Overcast days, don't even talk about overcast days. Like supposedly they use this metric for cities across the U.S. like how many overcast days per year? But they do not grade the overcast days by intensity. Right? And so supposedly like Cleveland has like as many overcast days, whatever is Seattle. No, no. That is bullshit when you're on the ground. So yeah, when you live under that oppressive blanket, you're going to need as much caffeine as you can lay hands on. And this is why Seattle is a [crosstalk 01:22:19]. Denny Lee (01:22:19): Oh, yeah. Well that and also don't forget, we are also known for being a rainy city, but actually there are sections in Nevada that actually received more rain than us. Rob Collie (01:22:28): Well, in terms of inches of rain. Again, like I grew up in Orlando, there's more rain there too. Right. It's just that in Seattle, it falls in a constant mist forever. Denny Lee (01:22:37): But don't forget. That's why people like me love it because you got a Gore-Tex jacket, eh, whatever. We don't care, we just don't care. But back to the coffee thing, because you know, I'm going to OCD on you. Yes. I'm glad to, at any point in time, dive into all the particulars. Rob Collie (01:22:58): We want to have you back on for sure. And we're going to make time to talk about your coffee... What am I going to call it? Like your rig? We need to talk about what your set up is like we're talking to The Edge from U2 about his guitar affects, right? We need to know. Denny Lee (01:23:16): You got it, dude. You know full well it'll be pretty easy to convince me to talk about data or coffee. So that's what we're going to do. Rob Collie (01:23:24): Seriously. If you're open to it, I'd love to do this together because there's so many things we talked about that we didn't have a chance to like really explore. Denny Lee (01:23:30): We left the wording about dynamic structured cache. I still haven't addressed that. The reason why I'm saying I agree with structures because that's the whole point. The data that comes in may not be structured, but just to your point, when I want to create, when I want to make sense, I want to see those rectangles. I don't want to see a bunch of circles, stars, triangles. I need those rectangles so I can actually do something with that data. That's what it boils down to. Whether it's Coriant processing ETL, machine learning. I don't care. I just need to be able to do some of that. There has to be a structure to that data first before I can do anything with it. So the structure agree. The reason I'm saying cache, I don't agree with completely is because the point is, cache is meant as when you hit a final state and you're trying to improve performance. Denny Lee (01:24:20): This is where cache is actually extremely beneficial. I'm not against caches, by the way, I'm just saying, but the reason why I'm saying it can't be a cache is because you're going to do something with that data from its original state to getting to a structured state. And then in fact, you may go ahead and do more things, standard part of ETL process. If you still remember our old data warehousing days where we have the old TP transactional database that goes into a staging database that goes into data warehouse before it goes into [NOLA 01:24:49] queue, right? It's analogous to this concept of data quality, right? The data is dirtier in the bating, or at least not structured the way for purpose of analysis at the beginning and overtime from [inaudible 01:25:01] TP to staging to data warehousing, it gets closer to a format or structure that is beneficial for people to query and to ask questions, right? Denny Lee (01:25:09): The same thing for a Data Lake. Often we talk about it as the Delta medallion architecture, but it's a data quality framework. The bronze silver gold concept. Bronze is when the data is garbage in garbage out silver's when I do the augmentation and transformation of it, gold is when it's proper feature engineering for machine learning or aggregate format for BI queries. Okay. But irrelevant of how I define or what wording I use, that's how to cache. I have to put that data down in state for the same reason I had to do it with LTP staging data, where I was the biggest. How about if there had to be a change upstream? If there's a change to the [LTP 01:25:44] database, I need to reflect that to staging and data warehousing. If I don't have the original LTP data, I can't go ahead and reflect that into the staging and data warehousing. Denny Lee (01:25:52): If I need to change the business logic, I need to go back to the original TP source so I can change it downstream into your staging, into your data warehouse. Same concept with the Data Lake. I'm going to need to go back to the original data based on new business requirements and reflect that change. So that's why it's not a cache because I need it stateful so I can do something with it. Ultimately, you want to make sure as the Power BI pro, the data that you're showing to your end users is as correct as you could possibly be. So whether it's technology or process, you're going to need both to ensure that, and this is what we've been discussing today is the ability for your Data Lakes to have that now. Rob Collie (01:26:40): All right, this is so good. I'm glad we had this [inaudible 01:26:43] I'm glad we made the time. Thank you so much. Denny Lee (01:26:44): Thanks buddy. Announcer (01:26:45): Thanks for listening to The Raw Data by P3 Adaptive podcast. Let the experts at P3 Adaptive help your business. Just go to P3adaptive.com. Have a data day.