The power of error analysis, tree models for search relevancy, what ChatGPT means for data scientists - Sergey Feldman - The Data Scientist Show #059
The Data Scientist Show - Daliana Liu - A podcast by Daliana Liu
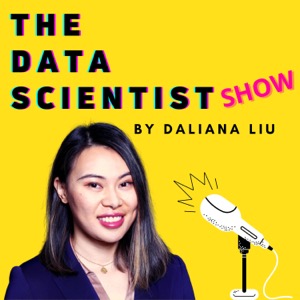
Categories:
Sergey Feldman is the head of AI at Alongside, providing mental health support for students. He is also a Lead Applied Research Scientist at Allen Institute for AI, where he built an ML model that improved search relevancy for scientific literature. Sergey has a PhD in Electrical and Electronics Engineering from the University of Washington. Today we’ll talk about machine learning for search, his consulting project for the Gates Foundation, AI for mental health, and career lessons. Make sure you listen till the end. If you like the show, subscribe, leave a comment, and give us a 5-star review. Subscribe to Daliana's newsletter on www.dalianaliu.com/ for more on data science. Daliana's Twitter: https://twitter.com/DalianaLiuDaliana's Daliana's LinkedIn: https://www.linkedin.com/in/dalianaliu/ Sergey's LinkedIn: https://www.linkedin.com/in/sergey-feldman-6b45074b/ Data Cowboys: http://www.data-cowboys.com/ Sergey Feldman: You Should Probably Be Doing Nested Cross-Validation | PyData Miami 2019: https://www.youtube.com/watch?v=DuDtXtKNpZs December 4th, 2018 - Breakfast with WACh with Dr. Sergey Feldman, PhD: https://www.youtube.com/watch?v=vA_czRcCpvQ (00:00:00) Introduction (00:01:24) Machine learning skeptic (00:03:02) Tree-based models for search relevance (00:14:34) How to do error analysis (00:19:20) Nested cross-validation (00:21:34) Model evaluation (00:30:43) Error analysis common mistakes (00:33:37) How to avoid overfitting (00:35:56) Consulting project with Gates Foundation (00:41:16) Tree-based models vs linear models (00:45:19) Working with non-tech stakeholders (00:50:20) Chatbot for teen’s mental health (00:54:32) Can ChatGPT provide therapy? (00:58:12) How he got into machine learning (01:02:12) How to not have a boss (01:03:46) Feelings vs Facts (01:09:02) Future of machine learning (01:11:30) How to prepare for the future (01:13:39) AutoML (01:17:12) His passion for large language models