Stop Feeding Garbage Data To Your ML Models, Clean It Up With Galileo
AI Engineering Podcast - A podcast by Tobias Macey
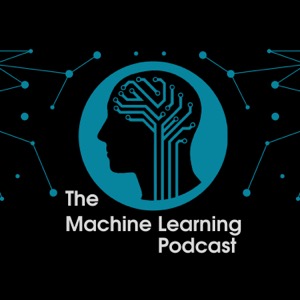
Categories:
SummaryMachine learning is a force multiplier that can generate an outsized impact on your organization. Unfortunately, if you are feeding your ML model garbage data, then you will get orders of magnitude more garbage out of it. The team behind Galileo experienced that pain for themselves and have set out to make data management and cleaning for machine learning a first class concern in your workflow. In this episode Vikram Chatterji shares the story of how Galileo got started and how you can use their platform to fix your ML data so that you can get back to the fun parts.AnnouncementsHello and welcome to the Machine Learning Podcast, the podcast about machine learning and how to bring it from idea to delivery.Predibase is a low-code ML platform without low-code limits. Built on top of our open source foundations of Ludwig and Horovod, our platform allows you to train state-of-the-art ML and deep learning models on your datasets at scale. Our platform works on text, images, tabular, audio and multi-modal data using our novel compositional model architecture. We allow users to operationalize models on top of the modern data stack, through REST and PQL – an extension of SQL that puts predictive power in the hands of data practitioners. Go to themachinelearningpodcast.com/predibase today to learn more and try it out!Do you wish you could use artificial intelligence to drive your business the way Big Tech does, but don’t have a money printer? Graft is a cloud-native platform that aims to make the AI of the 1% accessible to the 99%. Wield the most advanced techniques for unlocking the value of data, including text, images, video, audio, and graphs. No machine learning skills required, no team to hire, and no infrastructure to build or maintain. For more information on Graft or to schedule a demo, visit themachinelearningpodcast.com/graft today and tell them Tobias sent you.Building good ML models is hard, but testing them properly is even harder. At Deepchecks, they built an open-source testing framework that follows best practices, ensuring that your models behave as expected. Get started quickly using their built-in library of checks for testing and validating your model’s behavior and performance, and extend it to meet your specific needs as your model evolves. Accelerate your machine learning projects by building trust in your models and automating the testing that you used to do manually. Go to themachinelearningpodcast.com/deepchecks today to get started!Your host is Tobias Macey and today I’m interviewing Vikram Chatterji about Galileo, a platform for uncovering and addressing data problems to improve your model qualityInterviewIntroductionHow did you get involved in machine learning?Can you describe what Galileo is and the story behind it?Who are the target users of the platform and what are the tools/workflows that you are replacing? How does that focus inform and influence the design and prioritization of features in the platform?What are some of the real-world impacts that you have experienced as a result of the kinds of data problems that you are addressing with Galileo?Can you describe how the Galileo product is implemented? What are some of the assumptions that you had formed from your own experiences that have been challenged as you worked with early design partners?The toolchains and model architectures of any given team is unlikely to be a perfect match across departments or organizations. What are the core principles/concepts that you have hooked into in order to provide the broadest compatibility? What are the model types/frameworks/etc. that you have had to forego support for in the early versions of your product?Can you describe the workflow for someone building a machine learning model and how Galileo fits across the various stages of that cycle? What are some of the biggest difficulties posed by the non-linear nature of the experimentation cycle in model development?What are some of the ways that you work to quantify the impact of your tool on the productivity and profit contributions of an ML team/organization?What are the most interesting, innovative, or unexpected ways that you have seen Galileo used?What are the most interesting, unexpected, or challenging lessons that you have learned while working on Galileo?When is Galileo the wrong choice?What do you have planned for the future of Galileo?Contact InfoLinkedInParting QuestionFrom your perspective, what is the biggest barrier to adoption of machine learning today?Closing AnnouncementsThank you for listening! Don’t forget to check out our other shows. The Data Engineering Podcast covers the latest on modern data management. Podcast.__init__ covers the Python language, its community, and the innovative ways it is being used.Visit the site to subscribe to the show, sign up for the mailing list, and read the show notes.If you’ve learned something or tried out a project from the show then tell us about it! Email [email protected]) with your story.To help other people find the show please leave a review on iTunes and tell your friends and co-workersLinksGalileoF1 ScoreTensorflowKerasSpaCyPodcast.__init__ EpisodePytorchPodcast.__init__ EpisodeMXNetJaxThe intro and outro music is from Hitman’s Lovesong feat. Paola Graziano by The Freak Fandango Orchestra/CC BY-SA 3.0