The Secret Sauce Behind Roofstock's Neighborhood Scores
The SFR Show - A podcast by Roofstock
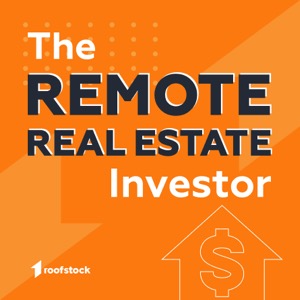
Categories:
If you have ever browsed the Roofstock Marketplace, you will be familiar with the neighborhood scores used for risk assessment. If you have ever wondered what goes into the calculation of these scores, join Tom and Michael as they interview the Head Data Scientist at Roofstock, Mike Polyakov, about what exactly goes into these values. --- Transcript Tom: Greetings, and welcome to the remote real estate investor. On today we have a special guest, Mike Polyakov, who is the head data scientist at Roofstock. And in this episode, we're going to be talking about the Roofstock neighborhood score. What goes into it? How is it updated? And what makes it special? All right, let's do it. Tom: Mike, thank you so much for coming on to the episode. You are the lead data scientists at Roofstock. Mike That's correct. Oh, yeah. Happy to be here. Tom: Excellent. So before we get into the specifics of the episode, which is going to be on the neighborhood score, I'd love to learn a little bit more about yourself before you got to Roofstock. What were you doing? And then now that you're being at Rootstock for a little bit of time, what's your kind of day to day like, like, so let's start at the beginning. What were you doing before you came to Rootstock and to be the lead data scientist? Mike: Sure. I have kind of an unusual background. So which combines political science. I have a PhD in political science from Berkeley, which I got in 2014 in computer science, which I guess, which is the kind of before a PhD, and then sort of went back after I finished the PhD, right before coming to Rootstock. I worked at crowd pack, which is a political crowdfunding startup, I believe they're still going. And there's some different leadership there in San Francisco, I was there for almost three years. Also doing data science there, since I joined Roofstock in 2017, worked on a variety of projects. Some of it is kind of typical data science things. So things like analyzing users trying to understand accorded the best leads, doing a bit of marketing work, and also more Roofstock specific things. So things like estimating rents, valuating variations of properties, and of course, the neighborhood score that we'll talk about here. Tom: Super interesting. Michael: Well, this is gonna get so off the rails so quickly. I mean, I would love to know what a PhD you know, what, what in most your classmates do after getting their PhDs, Mike: So it's going to be like a Stuff You Should Know. So it really varies. A lot of them actually stayed in academia in political science. One guy from my class went to back to Singapore, where he was from, and he's kind of a middle level bureaucrat there, some folks have teaching jobs, others just went back into the world and two totally random things. Tom: What brought you to getting into FinTech? Mike: It wasn't FinTech specifically, but that summer 2017 crowd pack was, you know, a little bit on the rocks. And I was looking around, and I was actually interested in investing in real estate, didn't know a whole lot about it had invested at that point, and kind of find out about rootstock through one of my alumni connections, and it seemed like a perfect opportunity. Tom: What better way to to learn than just jumping right in? Go ahead, Michael. Michael: Yeah, exactly. Yeah, I was gonna ask Mike, since learning about it, have you then since started investing in real estate? Mike: Yeah. So I'm a little embarrassed to say that for the last year and a half, I've been in sort of analysis paralysis, where I've been wanting to, but our sport is the market selection for me. I've done the Academy of both these most of the lectures. So I'm all ready to go except I need to start. Michael: Yeah, anytime you want. we'll hop on a coaching call. And we can talk through some of that analysis paralysis. Mike: Sounds great, man. Tom: Excellent. Excellent. Michael: We could go on forever, I'm sure. But let's talk about the neighborhood score, Tom. Tom: I know, I know. So first, I have a couple questions related to the neighborhood score. Let's start out with what are the different variables involved with it? And, you know, actually, I'm going to even take a step further back. Is there a general thesis of the neighborhood score of what we're trying to solve for? And how did it like internally on the data science team? What do you what is like the kind of the overarching goal when you think of the neighborhood score? Mike: Yeah, absolutely. It's best to start at the beginning. Yeah. So in the real estate world, and you've probably touched on this in some of the lectures, there's this notion of a neighborhood class, right, you might assign letter grades ABCD a being the best. And from investor's point of view, this is the mechanism to account for risk associated with location, right, so that for an investment, you can compare returns versus the versus the rest. Typically what those letter grades capture is both operational risk and the expectation, appreciation or decline of an area. And operational risk includes things like turnover evictions, effective age, rents, vacancy, all that stuff. The downside of that traditional neighborhood class notion is that one, there's no formal definition, right? It's kind of I know, when I see it sort of thing. And so when investors see might be not going to speed will vary even within the same market. But the other big issue is scale. Right. So most investors are focused on a single market. And so they lack national perspective, right, they might be assigned, might be able to assign some very accurate grade, so to speak with an Atlanta weather base, but really struggle to do the same thing as Charlotte. And so what the neighborhood score tends to do is to serve, operationalize it, make it scalable across the country, and use data to make it objective. So specifically for Rootstock neighborhood score, the goal is still to assign location based risk to properties, and specifically operational risk. So that's the start. Another important thing to say is kind of at the outset is what is neighborhood mean, for us, right? Because it's very fuzzy term. People mean different things when they say neighborhood, in our case, neighborhoods pretty large. Specifically, it's the census tract. So the US Census divides the entire country into tracks. And each track should be roughly the same number of households, it's about 1500. In a metro area like Atlanta, it's going to be comparable to a zip code. So you know, it's not going to be your block, or what some people might sort of colloquially refer to a neighborhood. So a little larger than that, but it allows us to get a lot of statistical power when we look at the data. And so what data do we use a lot of is actually what would be the same as what the real estate professionals would looked at. So it's things like information about the housing stock, but the individuals in the area, but the households, school scores are going to be pretty important and crime data, high level that that's what goes into score. Tom: Got it and on the size of the area. So you had mentioned like the census track is kind of a moving target based on how dense the area. Am I understanding that correctly? Mike: Well, no. So the idea of a census tract is that it should be roughly the same population. So any track Yeah, they're not gonna be exactly equal, but they're gonna be pretty similar. Tom: Does it relate to zip codes, or zip code plus two, or zip code plus four? And, and what does that mean zip code plus two plus, plus four? Mike: Yeah, so it doesn't, they're completely separate in all ways, except that in certain areas, there will be roughly comparable size, like in Atlanta, I just happen to know that a lot of the zip codes are about the same geographical size as the census tract when you say a zip code plus two, which is pretty uncommon, zip plus 4 is a little more common. So the USPS separates, basically cuts up any given zip codes into these little areas. And simple plus four is basically a nine digit number. It's your five digit zip code plus four more digits, which usually identify your specific block. So it's block level, geographic region. Tom: I'm already learning things. I always thought the neighborhood score was related to the zip at some level. So already as an employee, since for a very long time learning some stuff about the neighborhood score, and belaboring the point, but the size of the neighborhood is based on the census tract. Mike: Well, it is the census, it is the census track. Tom: Okay, got it. Yeah. You probably said that two times. Mike: It's just yeah, I mean, you know, for sure, the simple reason for that is, that's where most of the data, most of the reuse is assigned at that level. Right. So most of our inputs come from the US Census. And they usually deliver it in multiple levels. So you could also get some of these inputs at census block level, which is literally your street block, but it's much more sparse, and it's much less exact. So a good balance of kind of precision. And also coverage is at the census tract level. Michael: And Mike you touch on something there that I want to circle back to and make sure that I heard you right. In our listeners, we clarify for our listeners, did you say that the information that's going into the algorithm that builds the neighborhood score is coming actually from US Census. Mike: Not all of it. So most of it comes from census. The other components are a school scores, which we get from a vendor and crime data which we get from different vendors. Michael: Okay. So I think that's a question I get, oftentimes in the academy is where is this information coming from? Is it Zillow? Is it Redfin? Is it? Is it individually collected? So that's really interesting now that a lot of it comes from from the census itself. Mike: Yep. Tom: So we've had the neighborhood score out for a couple of years, has the waiting in the way that we weight different variables that go into it changed it all over time? And is there this kind of concept of like, I don't know, is it learning and getting smarter over time, I guess is another way to put it. Mike: Yeah. So it's a great question. Michael: It’s becoming sentient. Mike: Yeah. Tom: I hope not just we have to worry about AI and what's what's it called? Mike: The singularity. Tom Yeah. Mike: Not at Roofstock, it won’t happen here first. So that's a good question. And I think a lot of people have that question of, you know, how do we come up with the weights? And so I'm going to kick out a little bit. I'll try to keep it high level. Tom: Geek out away, geek out a little bit. And Michael, and I will raise our hands when we're drowning. Mike: Yeah, no, it's fine. It's fine. So the neighborhood score is not a supervised learning model, which means that so for a lot of models in AI and machine learning, generally, they're supervised in the sense that you have a training set that's labeled, right. So if you think about training model to recognize hot dogs, you have a bunch of pictures which are labeled hot dog or not hot dog right. That's your label training data set. You're trying to get the model to learn something that you can sort of look at and know immediately, right, because we know how to do this, right? So you try and get them all to replicate something that you know how to do it when that score is an unsupervised model in sense that while So, you could imagine having a professional, you know, going through 100 or 1000 neighborhoods and saying this is A this is B this is C you could approach it that way. What we chose to do instead is to say, look, this is the data that we know should determine the quality of this neighborhood. This is the inputs that I mentioned, we apply a process, that's known as dimensionality reduction, which takes all these different inputs, and then extracts a single number out of them. And the way it works is that imagine going to a doctor and getting your temperature measured, and maybe your heart rate measures, maybe your weight. And you can imagine all those measurements, giving you sort of an overall health score. Right? So having all those numbers, the doctor can say, Are you really good health, you create a health, or maybe not quite so well, maybe you're a grade B or C. The idea being that there's some underlying, sort of not objectively real, but an intuitive notion of health of a person that can be measured in these different signals. The same thing works for neighborhood score, you can imagine there's a kind of underlying quality of neighborhoods, which we're trying to get by these different measurements, looking at the school scores looking at, you know, household incomes, or percent owner occupied homes. These are all individual measures, which we combine them we can extract an overall quality, if that makes sense. Michael: That makes total sense. And such a great way of explaining it. As a total side note, tangent there actually isn't this app, it's called I think fingers are hot dogs. And you like hold your fingers up, and it has the apple guess whether if they think it's a finger or a hot dog? Mike: Yeah, well, that's from Silicon Valley, right? Michael: Yeah. Tom: Like Michael said, you did a really good job, like talking about the concept of unsupervised data versus supervised data in kind of understanding and how it is evolving in that way. So on the notion of evolving, how often are the variables that go into it being updated? Mike: Right, so to get to the more precise career question or that part, so the data itself changes on various time scales. So the US Census releases their data every year, and we're using the American Community Survey, which is part of the US Census, and they redo the survey every year. So that's updated annually, the schools personally, updated monthly, right now, for various reasons, we haven't been updating the score very much. What's important to know is that we've done some analysis to see how how much you would change year to year. And it's actually very little. So to give you a sense, from one year to another, I think less than 5% of census tracts, which change half a star or more. So most of them are quite stable. Michael: And kind of getting back to Tom's question a little bit, Mike, the weighting of the different factors that go into it. Can you talk to us a little bit about how that looks? Mike: Yeah. So the reason I brought up the unsupervised learning bit of it, it's that the weights are learned by the model? Well, so I think the back is, I wouldn't say that they're learned by the model, but they're assigned by the model. In other words, when the model looks at all the inputs, so going back to the doctor analogy, right, so maybe your your heart rate and your weight, and I don't know what what's another, another thing that they measure, blood pressure. Yeah, so maybe all of those are kind of pointing in one direction. So they're all correlated, but then your temperature is really low, unexpectedly low. So there's something going on that the other signals aren't picking up, but temperatures picking up really strongly. So what the model would do in that case, it would assign greater weight to the temperature than to the individual other inputs, because it thinks that temperature is showing you something that's not present in the other three signals. So in other words, if you have those four measures adopted, you could say that there is kind of two separate things going on in your body. One of them is picked out by heart rate, blood pressure, weight, and one of them is picked out by temperature. Interesting. So similarly, with the real estate case. So we don't want all those inputs. And I think there's nine, nine or 10 different inputs, the ones that have sort of more information than the others, like more distinct information is going to weigh those higher. So given that the inputs don't change very much here a year, the weightings aren't going to change very much year to year. Michael: But so, in theory, or maybe in reality, we could have different weightings for different markets based on the data set that's being provided. Mike: Um, so yes, we could so one step that I didn't mentioned this kind of the Emperor script before, once we collect the inputs from these different sources, we do some normalization to the values across markets. So that I mean, what you want ultimately, in your score, is for it to mean the same thing in different markets. So for certain planet, in terms of things you care about, like all the operational risk factors I mentioned before, so a 4 star in Atlanta should be similar to 4 star in Rochester, New York. And to allow that to happen. We do some normalization inputs before we run the model on. Michael: So that way you You shouldn't end up with a situation where a Atlanta market is more heavily weighted towards crime versus your neighborhood score. And Rochester is more heavily weighted towards, I don't know, appreciation potential, something like that. Mike: Yeah, that's sort of handled in the pre processing stage. Mike: Got it. Tom: If I was to look at all of the properties that have a neighborhood score wouldn't form like a bell curve where the majority of them are in the middle like this three star in just a few of them have five star and very few have one star, how is this kind of the shape? If you looked at the full data set, look at me sending like a data scientist? Michael: Great question. Mike: And yeah, that's a great question, Tom. Right on? Um, yeah, so it's actually it's a slightly right shifted bell curve. So what you find is that about a quarter of properties in the country, or single family homes are less than three stars, about a third, or three, three and a half stars. And the remainder, which is a little more than a third is going to be four stars and above. So it looks kind of like a bell curve, but it's a little bit shifted off center to the right. Michael: And is that properties in the nation or properties on Roofstock? Mike: Properties in nation? Michael: Wow, what about properties on Roofstock? Do we know what the data set looks like there? R Mike: Roofstock have a look at the curve recently. But it tends to be a little bit more left shifted? I think our me, our average is probably a little less than three, or maybe three, Michael: Which makes sense, because those are cash flowing properties. Tom: So my less last question for you is, how do you see the neighborhood? And do you see it evolving over time? Like, is there a roadmap for ways that we're working with the neighborhood in the future? I'd love to hear your input? Mike: Yeah, absolutely. So there's still like significant issues with the current input score. One is that we do have some areas which don't have any score at all. And this happens, because some of the inputs are missing. Sometimes it's from the census, we don't have a value for given track. Sometimes there's no school scores at the track level. So we're using, we're doing some work right now, to address this by improving our statistical methods, it should be more complete. In the near future. The other kind of issue more visible probably to the to the user browsing a website is going to be that you're coming back to this idea of neighbors corners being at the census tract level, that's a really pretty big region, right. So it's a very coarse scoring. And that also means you can have sharp boundaries. So it's not unusual to have with a two star neighborhood, adjoining a forced neighborhood, which, you know, looking at the census tract level, it may be fine. But around the border, there's likely going to be some inaccuracy. So if you pick up a property that's close to that border, but it's on the 2 star side, it's likely going to be a little bit in terms of separations, it's likely going to be a little bit more like a three star and vice versa. And so we're our next step, which you know, because for a while, but may actually happen next year, is we're going to move down in geographic granularity down to census block group level, which is a division of a track, it's about a one third of the size of a census tract. So it's not a huge improvement, but it's going to be helpful. And then we have some other things that we're going to do to address this short boundary issue. Tom: Excellent. Michael, do you have any any final questions for Mike? Michael: No, I mean, Mike gave me the punch, I was going to ask how folks should be thinking about or working around markets that have kind of a block by block change, where you know, you have a really good block and a really rough block. But I think the answer to that question kind of addressed it, and that it's going to be up and coming. But maybe add on maybe the question is still relevant? How should folks be thinking about and evaluating properties in those neighborhoods that really are sparked by block or street by street changing? Mike: Um, yeah, so a good rule of thumb. And actually, Tom can probably chime in on this as well. But a good rule of thumb is to look at rents. So at least within say, census tract, rent is going to be a pretty strong predictor of what actually sorry, so rent over price. So if you like, go on Zillow, and you look at the rent for property, and then it's so surprised, you can figure out the yield. And so within at tract properties that are more high yielding, will tend to have lower neighborhood scores. So for example, you know, you got a whole tract, that's a three star and then on the right, maybe it's closer to the highway. And you see, there's kind of like, if you look at a couple homes, that you kind of see a pattern of higher yields than the rest of the track, that's probably a slightly worse area. Michael: That makes total sense. So Mike, I'm curious to know, because on Roofstock, we have the neighborhood score in stars. And then we also have the school score as its own category in stars as well. But you mentioned that the school score is actually one of the inputs into the neighborhood score. So just curious why we have separate and distinct call outs. And, you know, why is the school score included in the neighborhood score, and also on its own called out? Mike: Yes, another great question. So it's including the input score, because, well, it's an important input, right? It's important signal of the socio economic index, which is what sort of neighbors score is, right? It's not necessarily entirely separate. So for example, if you took out school scores, and you kept all the other inputs, most scores won't change very much. So it's not contributing a whole lot of information. But it is useful as to why if we have the score, why do we have a separate school score? I think probably two reasons. One, I think people just have a very strong intuition that they want to look at school scores in an area, right? That's just information they want to see. And then it does in search for certain buyers, depending your investment thesis, it provides information that's not so relevant or not really communicate, but neighborhood score. So for example, you know, if you have a family, or if you want to rent to families, school scores are going to be probably more important than if you want to rent to young professionals. Michael: It makes total sense. Tom: My last question, not necessarily neighborhood related, talking about some of the other projects that you're excited about that or the data science team is in science is excited about anything, any specific project that's you think particularly interesting that you're working on right now? Outside the neighborhood? Mike: Yeah. Well, I'm hesitating because I'm sure, like any intellectual property, or what I'm trying to figure out, like, what yeah, exactly what what I should be revealing here. But I'll tell you one thing that's, you know, definitely not controversial. I think right now, we're not doing a great job helping people understand markets. I know because I need some help understanding markets. So we do have some work going on. In that respect. Some of it is more than short term. I think in the next month or two, we're going to have some market pages with better information, you know, it's going to help people make those choices and further down the road. Expect we're going to do more work on more machine learning and forecasting to help people understand markets now just as they are now but where they're going and how to think about that. Tom: Beautiful. Awesome, Michael, any final questions from you? Michael: No, this was super insightful. Mike, I kind of have my mind blown. This is this was awesome. Tom: I know, we got to have another episode and got editor, PhD political science. Mike: Yes. Absolutely. Michael: Want to both sides of the science, the political science, the meeting of the minds. Mike: Yeah, totally. Tom: Very cool. Well, thank you so much for coming on. And I have a feeling we'll probably be asking you to jump on again in the in the near future. super interesting. Michael: Great stuff. Mike: Yeah. Anytime. My pleasure. Thanks, Tom. Tom: Thanks, Mike. Michael: Thanks, Mike. Tom: Thank you so much to Mike for coming on today and telling us about the neighborhood score and a little bit about his background, looking forward to having him on again in the future. And if you like this podcast, like this episode, we would love it if you would subscribe, give us a rating, and all of that good stuff. All right. Happy investing.