Retrieving Texts based on Abstract Descriptions Explained!
Weaviate Podcast - A podcast by Weaviate
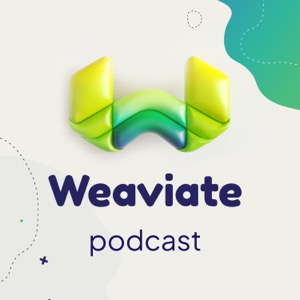
Categories:
This video explores a new paper exploring the use of summarization chains to represent long texts and use (original text, summary) pairs for optimizing text embeddings models! Here are 3 main takeaways I think everyone working with Weaviate may get value from: 1. Understanding of Summary Indexing and the Prompts (as well as Prompt Chains) used to build them. 2. Continued development of LLM-generated data for search -- creating (full text, summary) pairs gives you (1) data to build a summary index with as mentioned, (2) data to compare different embedding models with, and (3) data to train your own embedding model. 3. Tournament style evaluation with human annotators -- the top 5 retrieved texts from one model are concatenated with the top 5 from another model, these 10 are given to human annotators to pick 5 and this is how the authors are reporting the performance of their models rather than traditional benchmarks. This m ay be a more productive evaluation technique for most real world search applications. Thank you so much for watching, here are some links mentioned in the video! Retrieving Texts based on Abstract Descriptions: https://arxiv.org/abs/2305.12517 Weaviate Blog - Combining LangChain and Weaviate: https://weaviate.io/blog/combining-langchain-and-weaviate Weaviate Blog - Generative Feedback Loops: https://weaviate.io/blog/generative-feedback-loops-with-llms Jerry Liu in Llama Index Blog - A New Document Summary Index for LLM-powered QA Systems: https://medium.com/llamaindex-blog/a-new-document-summary-index-for-llm-powered-qa-systems-9a32ece2f9ec Learning to Retrieve Passages without Supervision (Spider): https://arxiv.org/pdf/2112.07708.pdf Weaviate Blog - Analysis of Spider - https://weaviate.io/blog/research-insights-spider Chapters 0:00 Introduction 0:13 Quick Overview 7:30 How to use in Weaviate! 7:50 Background 12:08 Motivation 14:20 Prompts Used 18:14 More Details of training 21:12 Human Evaluation Study 22:40 My Takeaways from the Paper