Matt Sigelman: Navigating the Skills Landscape of the Future
Work Forces - A podcast by Work Forces - Tuesdays
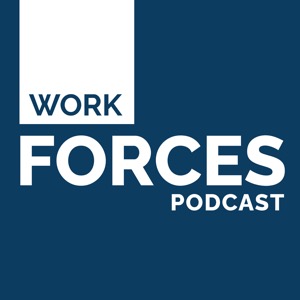
Matt Sigelman, President of the Burning Glass Institute, discusses the crucial role of real-time labor market data in guiding workforce development and helping stakeholders navigate the evolving skills landscape. Sigelman highlights the rapid evolution of skills, noting the decreasing 'half-life' of skills and the growing importance of lifelong learning. The episode explores how workforce systems can adapt to these changes, including the need for better training infrastructure and a focus on career progression. Sigelman also examines the impact of artificial intelligence (AI) on the future of work and the importance of complementing AI with human skills to ensure a more equitable and prosperous future. Transcript Julian Alssid: Welcome to Work Forces. I'm Julian Alssid. Kaitlin LeMoine: And I'm Kaitlin LeMoine, and we speak with the innovators who shape the future of work and learning. Julian Alssid: Together, we unpack the complex elements of workforce and career preparation and offer practical solutions that can be scaled and sustained. Kaitlin LeMoine: Work Forces is supported by Lumina Foundation. Lumina is an independent, private foundation in Indianapolis that is committed to making opportunities for learning beyond high school available to all. Let's dive in. So Julian, for years, we've been helping clients navigate the messy intersection of work and learning, and using and interpreting labor market data has been essential to help inform and guide the direction and decision making of various stakeholders, ranging from business and industry to higher ed and training providers. Julian Alssid: It's true Kaitlin, but early on, data tools were limited government data lags, which made it hard to keep up with pace of change, and the tools available were also challenging to use or interpret, which which made it difficult to adopt the use of this data across whole teams and organizations. Often, data analysis was housed under, you know, one person, or in a small analytics shop, which further like limited ongoing use by the individuals charged with educating and training and hiring. And to compound this all further, students and workers were even further removed from labor market data and related trends. Kaitlin LeMoine: And that's why the work of our guest today is so critical. Matt Sigelman, president of the Burning Glass Institute, has dedicated his career to unlocking new avenues for mobility, opportunity and equity through skills. He and his team created the field of real time labor market data, a breakthrough innovation which has transformed how we understand and navigate the world of work. Julian Alssid: Matt's work has cracked the code of an increasingly dynamic labor market tracking demand for 10s of 1000s of skills across 30 countries before before launching the Burning Glass Institute, he was CEO and then Chairman of Lightcast for over 20 years, and worked at McKinsey & Company and Capital One. Matt also currently serves as a senior advisor at the Harvard Project on the workforce, and is founder of the elementary school mainline classical Academy. Is it? He writes widely on the job market, and it's consulted frequently by public officials and the global media. Kaitlin LeMoine: Matt, welcome to Work Forces. We're so excited to have you with us today. Matt Sigelman: It's great to be together. Thanks so much for having me. Kaitlin LeMoine: Great. Well, so as we jump into the conversation today, can you please tell us a bit about your background and your current role at the Burning Glass Institute. Matt Sigelman: As you pointed out, I spent 20 years before starting the Burning Glass Institute, building what's now come to be called Lightcast. What a lot of people don't realize is that, like cast started not as a data company, but as a job matching company. We built a engine for first being able to read people's resumes, and then to be able to what they call resume parsing, and then to be able to match them to jobs. And it was a successful little business and we wound up doing a lot of work in state and local workforce systems, providing them the the matching engines that were used to be able to support clients and connecting them with work. And this was around kind of the start of the Great Recession, when suddenly a lot of workforce agencies found themselves inundated with people, many of whom weren't traditional kinds of workforce system customers. People who are professionals or from a wide array of occupations and, you know, we had this great engine and, and what we realized, though, is that it could only work well if it could connect people with jobs. There had to be jobs for them to be capable, to be connected to and what, of course, your listeners who work in workforce systems know is that employers haven't always been so forthcoming in connecting and putting their jobs in front of workforce systems. And so what we essentially had was this Ferrari engine flight plucked down inside of a Yugo. Right, you know, it searched across the 100 jobs that happened to be listed on, on what effectively was an online bulletin board in, you know, in a workforce center. And so what occurred to us is that we were solving the wrong problem. We were solving a one on one matching problem. That is to say, how do I match Matt with a specific job? But so much of the problem that we have in workforce development, economic development, in policy, but also in the world, of education and employers? Well, is a planning problem. It's not a one on one matching. It's a problem. It's it's a how do it's a it's a population genetics problem, right? Like, how do you connect this group of people with these kinds of jobs? Because that's when you can start to plan. And so we started scratching our heads and said, Why is that not happening? And what we realized is the same reason why our matching engine was constrained. The fact that there weren't a lot of jobs in one place was the same reason that a lot of that planning wasn't happening. And so we said, Well, gee, this is this is strange right now. Pretty much most job postings. And I think this was largely true, then it's certainly a lot more true 17 years later today, that pretty much most job postings across whether you're talking about very entry level jobs, whether you're talking about highly skilled professional jobs, are posted online. And so instead of having to rely on employers to come to you, you could actually go and just scrape their jobs. And so we we built a whole system of spiders to crawl the web and bring back what ultimately became millions of job postings every day. But our ambition, again, was, was not so much to create a bigger database for a matching service. Our ambition really quickly became to be able to say, what do those jobs say? How can they help us plan more effectively, so that we're not leaving people, leaving training, leaving policy at the mercy of the spot market, where it's like, okay, what jobs happen to be available today? But instead to be able to think ahead and say, Hey, what's the training that we're gonna need to be what are the programs that we're gonna need to invest in? So, what we did is we started to develop all these coding engines to be able to make sense and interpret, make sense of and interpret all the jobs that we're bringing back. And that was sort of the birth of what people have come to refer to as the real time labor market industry. And it's, it's evolved greatly since then, and I'm proud of the work that Lightcast and others are doing, to continue to advance those data. I left that three years ago, and I left it because as powerful as the data are, I came to realize that a lot of what's missing is the ability, is, the trend is, is the translation and transformation layers? How do we use these data to affect fundamental transformations? And a lot of that really isn't necessarily about the data themselves. It's not about software, but it's about working together with stakeholders in the field and solving things very experimentally, because a lot of these big problems, the big, thorny problems that we're all trying to solve, well, inherently, they haven't been solved yet. So the Institute is a fully independent nonprofit data laboratory to advance research and practice at the intersection of the of the future of work and the future of learning, where our goal is to undertake the kinds of experiments that advance economic mobility, that build community prosperity and that ensure that we're, we're seeing better outcomes for workers and learners. Julian Alssid: It's great Matt to hear you tell the story, and you know kind of I've, I've known you and really followed you through this, most all of this process, and I'm glad that you're now at the intersection of work and learning and trying to help solve these, these thorny problems. What? What are some of the trends that you're seeing now? And you know, at that intersection and in particular areas of research that you're really excited about and are kind of looking to further unpack. Matt Sigelman: So when I think about the intersection of work and learning, what's shaping the imperative there and shaping the greatest sets of transformations is the level of dynamism and skills. We're used to thinking about a labor market that's denominated by jobs and a lot of the prognostication that we see is about jobs going away, new jobs being born. What we see instead is that the greatest changes are happening at the layer of skills. What are the skills that it takes to land a job? What are the skills that get accrued within a job, and what's the dynamism that's reshaping those skills that's really important, because the skills affect not only what it takes to get a job, but they affect how you get your next job. You think about a job transition, you're essentially taking the skills that you just learned, and you're moving from job to job. And what we're seeing is a tremendous rate of skill change. People often talk about a half life of skills. Listeners won't be surprised to hear that that half life is quickening and quickening. Well, quickly, we did some work a few years ago with our colleagues at BCG, where we found that the average job has seen 37% of its skills replaced in just the prior five years, and that was before generative AI. So here's the thing about it, though, when we think about that pace of skill change, we really sort of assume it's about technology change, and there's certainly no small amount of that, but the greater set of changes are actually about skills blending from across domains. Think about a field like marketing. You know, we all know people in marketing, they tend to be very nice people because they went into marketing because they know how to talk to people well. So you have right brain people, and all of a sudden marketing is becoming a data driven sport. So you have right brain people who need to be able to use left brain skills, not hard to find marketing people, but try finding a marketing person with SQL skills, and the job becomes much harder to fill. It comes, you know, starts to command big salary premiums. So we're seeing that kind of phenomenon around the market. Here's why I talk about that as as the key imperative shaping the intersection of work and learning, because what it says is that a educational model of once and done learning. You go to high school, maybe you go to college, and then you sort of never look back and go a step into your career. A model like that that was already challenged is increasingly broken or perhaps risible at minimum. I think you could say it's inadequate. Maybe that was a more polite way of saying it so. What it says is that there's 165 million US workers in the workforce, and the vast majority of them are in a position where they need to be able to acquire new skills, and they need to be able to acquire new skills on the fly. And we need an infrastructure for that. The logical infrastructure that we have things like our workforce system is tremendously under invested. Think about the scope of Workforce Investment monies that actually get allocated towards skill training, talking about a few 100 million dollars out of a couple billion of total WIOA funds a year. You think about our community colleges, which are slightly better funded, but the vast majority of, or at least significant majority of community college enrollments right now are into we're called degree transfer programs and not to workforce training. And so we really are leaving people to navigate their transitions without much guidance and without the resources to acquire the learning that enables them to move up, that gives them a greater chance of making sure that each of those transition is an opportunity for greater economic mobility. The average US worker right now will see 12 career transitions over the course of her career, and each of those transitions is an opportunity to either move up or to stay put or perhaps even fall back. And right now, we are not helping people systematically to discover what are the skills that will help them move up? What are the skills that are in demand that will allow them to command a premium? What are the skills that will prepare them for the future? And even if we help them discover those skills, we don't provide them with an infrastructure to acquire them. So you know that says to me that there's a whole big space around around lifelong learning. It has implications for higher ed, because right now, higher ed has largely surrendered that space to online players, and I think there's a big opportunity for colleges and universities in a time that they're seeing enrollments thin. It also has implications for how we think about non degree credentials and what it takes to make them successful. Kaitlin LeMoine: I think that what you've said about kind of this moment of both the reality that the skills are changing very, very quickly, right? And what's needed as far as the half life of, kind of like, what's most up to date, as far as job relevant skills is shifting more and more quickly, kind of at the same moment as perhaps we're all needing to rethink, what does it look like to keep up to speed. I guess I'm wondering, like, who's whose job is this to figure this out, right? Because it feels like it's both on the it's, it maybe lives with the individual. It might live with employers. It certainly could live with higher ed and training institutions, but only if learners are going to them. So kind of, how do we think about, you know, how we all work to solve this challenge? It's a really, really big one that you're raising here. Matt Sigelman: So I'm so glad you raised this, this question. There's right now no shortage of dysfunction in the market. For the most part, what we have is a system in which the responsibility for navigation rests with individuals themselves, with individual workers and learners. And that's a problem because individual workers lack information, they lack counseling, and they lack liquidity, both financial liquidity and time liquidity. They lack the financial liquidity to be able to invest in training and the time liquidity to be able to have the time to do it when... Kaitlin LeMoine: Or even be aware that they need to do it right. Matt Sigelman: Exactly. And so if you sort of think about where there would be logic in this. There'd be a lot of logic in employers investing. There'd be a lot of logic in the public investing in this. But both are encumbered right now by issues of cost, time and risk. We've got employers who, whether logically or not, wind up getting obsessed with what economists call free rider problems, right? You know, I've got a workforce that's likely to turn over very quickly, and it's true that, you know, in a lot of roles, retention is historically low and and so you know, why should I, as an employer, invest in training somebody who's likely to be leaving now, there's, there's all sorts of good logic for why they should, but, but regardless, you can understand the perception of how it's hard to for an employer to get a return on investment when the time duration of their return is is likely to be so low. But it doesn't change the fact that employers, the ones who are in the best position to signal to their employer, to their employees, right? You know, you would want an employer to say, Hey, Matt, I'm struggling to fill these jobs up here, and I think you could fill them, I want to pay you more. Here's the learning that I want you to take on. Here's the training I'm going to invest in you to get. The public has significant stake in this as well as we think about, you know, kind of our modern Sputnik moments in our global contest for economic competitiveness, as we we try to navigate an increasingly fraught geopolitical environment, the ability to be able to have a highly skilled workforce working in the kind of sectors that we think are going to be most important means that we need to have the opportunities to invest. But here too, I think, you know, we're, we're struggling with issues of, of cost on the one side, of planning on the other, which is, which is a time question, how do we predict where those changes can be and what the sequence of those changes going to be? And so, as a result, what we have, and by the way, educators, likewise, are either asleep at the switch in the sense that they're not offering the kinds of programs that are oriented towards selling degrees. And they are also where they are trying to promote things. Are trying to promote their programs. They're self interested. Not surprisingly, I think here's where we can change this dysfunction. And been lucky to be collaborating with a group of of 30 people in in a project at Stanford right now being led by by Mitchell Stevens, where we're really trying to say, what would what the new economics of learning be, if you were trying to think about moving from an educated society where education happens at the start of a career to a learning society? Where learning happens throughout a career. I think you can imagine sets of structures that allow us to both reduce risk through more effective information sharing, through better signaling, but also to share risk. You can imagine financial structures, for example, where if employer invests in an employee and training employee and the employee leaves that the employer gets a tax credit, regardless of whether the employee is still at the firm, proportionate to the amount somebody's income rises, which, by the way, is something you could fund out of the greater tax revenues that you get when people are earning more. So there's structures that we could put in place that would allow us to reduce risk, having better Labor Market Information Systems, having better mechanisms for employers to share data on the training that they're investing into their workers. Having better assessments of credentials, are all mechanisms that we can put in place, and some of which, you know, we're trying to advance at the institute. Julian Alssid: I almost feel like we haven't even gotten to AI yet. So speaking of which, you know, as AI is sort of rapidly, you know, permeating all. How do you see, how do you see it impacting today and the future of work and learning, you know, short and long term, and, and, and, you know, and kind of the construct you're laying out for us. Matt Sigelman: So there's a couple of effects that we've been we've been studying one that I've been working on, together with, with Mike Fenlon, who was formerly the the Chief Human Resources Officer at PwC, and with Joe Fuller, professor at Harvard Business School, and a colleague on the Harvard Project on the Workforce. And what we've been looking at is how AI is going to reshape learning curves. It turns out, what we found from our research that a lot of the impacts that we've been sort of thinking about as impacts on on jobs or on tasks are really about how AI shifts expertise, how much expertise is required to get into a job, and how expertise gets accrued over the course of a job, essentially, what's the shape of a learning curve? And what we find is that there are some kinds of jobs where learning curves tend to be relatively flat, where there's a fair amount of skill that's required to get into a job, but once you get into a job, productivity remains fairly constant, no matter the level of experience that you accrue. Think about the job of a bus driver, for example. Maybe not a great example, because it's probably not going to be particularly impacted by AI, but just as an example of a job with a flat learning curve, those jobs are worth, by the way, sort of paying attention to, because those are jobs where the hypothesis that we sometimes hear about how AI is going to be the worker best friend, they're going to democratize knowledge. They're going to help overcome barriers to access. Those are the kinds of jobs where, where that's likely to be the case, where, essentially, you needed domain expertise to get in. You needed language proficiency, whatever it was. But you know, once you've got that, you can do that job. And so I think those are going to be places where we may, in fact, see AI reduce the level of expertise that's needed, but there's a lot of other jobs where what you see is a pretty steep learning curve over time. And what that says is that expertise is accrued over time, the productivity rises over time. And what you can then do is look at the differences in skills that are required at the entry level versus for somebody who's highly experienced in the domain and in many of those kinds of jobs. You see is the AI impacts are much more the AI overlaps are much more pronounced at the entry level. That says in big parts of the economy, the entry level opportunity landscape is going to dwindle. Think about what that means, both for people who are educating and training people, and what it means on the other side, for employers, for for educators, it says, what it mean to train people to start their careers in the middle, as opposed to at the beginning, when people are going to need that much more proficiency, when we're already struggling to make effective connections between education and work, and now we need to to raise the bar the. That's a critical challenge. On the other hand, think about what this means for an employer, where whole organizations right now are shaped around the idea that you hire a whole bunch of people on the entry level, and over time, that field narrows itself, and a few people get, you know, at each stage kept promoted up, and so you have organizational structures that are essentially pyramid in shape. Now of a sudden, you're thinking about diamond shaped organizations where you really don't need a lot of people at the entry level. Okay, fair enough. But how do you get talent when your whole pipeline has always depended on having lots of entry level people who are getting early experiences, and through those experiences becoming expert. So that's one of the AI impacts that we've been thinking a lot about. We have another branch of research we've been looking at, as you might imagine, we spend a lot of time thinking about skills, and so we've been trying to not only translate a lot of the the early models of AI impacts, which are about tasks, into skills, what are the, what's the capabilities that people need in order to do work in an AI era, but specifically, trying to intermediate this tug of war between, you know, are, is AI going to automate work or augment work? And so, you know, it's sort of like the the taste great, less filling of our age. I think the three of us are old enough to remember that. I don't know if our listeners are, but in any case, you know, there's been when you think about skills. Are, you know, are some of these skills going to get replaced, or is AI going to help us do them better? And so we've developed a set of data models to help us sort of think through this. And here's the fascinating thing, we approached this as trying to intermediate a tug of war. And a funny thing happened. What we found was that some of the same skills showed up atop both of our models. In other words, we were saying, Here's, you know, take a given skill and and it's, it's the top of most likely to to get automated away, and in top of the most likely to get augmented by AI. And so we, first we looked at, we're like, okay, we screwed something up royally, because, like, we were trying to, like, intermediate and exactly, and then, and then, when we looked into it, what we realized is there are sets of skills think about as an example data analysis, where AI increases our efficiency and it increases our effectiveness. And those kinds of skills are going to be a new power skills for the 21st century. And so we've been thinking a lot about, how do we make sure that everyone has those new power skills? Interestingly enough, even things like writing are among those new power skills. You know, the the exercise of writing looks very different in an AI era, but the ability to craft narrative, to shape logic, actually become more important, not less, because that's how we we leverage AI effectively so and then finally, as we think about AI, and it's both the disruptions that it portends, but also the opportunities that it opens, again, we've been thinking about skills. So if you start again with this idea that AI is going to change the skills that are required in a given job because some of the skills are no longer needed, other skills become more important. And then put that alongside something which I said earlier in our conversation, that the way right now, you get from job to job is by building on the skills that you've been using and bridging the gaps and using that to be able to to step across to a new job. Well, wait a second, if the skills that you're using in any given job are going to be different. What it means is that your next job, the transitions, the pathways by which people move from job job, actually get totally reshaped as well. Think about...give you an example right now, in the world of machining, you go from being a machinist to being a computer numerical control press operator to being a CNC programmer. Well, you can imagine that the distance between some of those may become, you know, for example, the distance from being a machinist to being a programmer may actually, you know, get compressed as CNC programming becomes easier to do. On the other hand, what that may mean is that a ladder that might have existed between CNC programming and actual software development and computer programming may get stretched for pulled further apart. So, you know, it doesn't mean that the transitions are no longer available, but there'll be different transitions, and so the kind of training that we've been used to providing to help people bridge gaps is going to need to be rethought. Kaitlin LeMoine: Circling back what you just said connects back to something I feel like that you had said earlier. And I was really intrigued by regarding, you know, this, this notion that, through AI really access, and frankly, I guess it's true of the internet in some cases too, just that the access to knowledge is ever present, right? Like the ability to rapidly obtain information about any topic is, it's kind of limitless. And what that means for that kind of diamond shaped job market, I think, is really fascinating, and it's what it's really intriguing to me. Like, what happens when knowledge might be ever present, but the skills needed to be engaging at that next level up are things like communication, conflict resolution, negotiation, right, skills that are perhaps that can't be honed with AI alone. And so what you just said about like the gap between, or how you bridge the gaps between these skills might look and feel really different. Just feels, I feel like that's where, where we're headed now is, what does it look like? And I don't have the answer, but this is one thing I've been wrestling with. Is, is, what does it look like to build training models and education models that help people, you know, kind of get to that next level a little earlier with those other skills, beyond the knowledge acquisition component. I don't know if that rings true to you, Matt, but that's just been on my mind. Matt Sigelman: Absolutely two things that I'll say there. You know, one, I've been thinking a lot recently about artificial intelligence and human intelligence, and how, in a lot of ways, they're almost exactly opposite each other. AI is, you know, what we're seeing with generative AI in particular is, is that Gen AI is, is phenomenally powerful as a pattern recognition engine, but and the human brain is a powerful human, you know, pattern recognition engine as well, but, but here's, here's why it's worth distinguishing them. Generative AI works by just sort of crunching tremendous amounts of data and looking for the patterns within those data. And so in a lot of ways, it's totally agnostic to content. It just crunching through it and seeing the relationships. And eventually, if you put enough into it, it can confine patterns and relationships. Again, human brain is a great pattern recognition machine as well. But think about your brain works. Your brain is not actually sort of chewing through every relationship that it could possibly look for. Your brain works exactly opposite. What we're really, really good at is filtering, is being able to very quickly, almost immediately, seeing the most salient relationships the human brain works by constructing narrative. I can give you a picture and you will immediately zoom in on the most salient thing in that picture, the thing that's that's most unusual, because you can see the story. And I think when we kind of see those kinds of differences, means understanding the difference between generative AI and and and human intelligence, we can understand how the two can complement each other, and what kinds of domains will be most complimentary. Kaitlin LeMoine: Yeah, no, that makes a lot of sense. Julian Alssid: It really feels a bit like what you're saying is we need to, we need to do what we do really well, but understand how to work with the technology. Matt Sigelman: That means as well that we need to have the ability. We need to have both sets of abilities. You know, I keep hearing something. I keep hearing people say, Well, gee, you know, we're not going to need to code anymore, because generative AI is going to do it for us. Where we're not going to do data analytics because the generative AI is going to do it for us. We will need fewer specialists, but actually, many more of us will need coding skills. Many more of us will need data analytics skills. The analogy I would offer here is, is Microsoft Excel, which, in a way, sort of automated some of the work that that, you know, kind of ledger accountants did and the like. Think about all the ways that we each use data today, whether it's to create charts, whether it's to, you know, kind of build a discounted cash flow analysis, whatever it may be, and think about what that would have been like to do without Excel. The answer is, you wouldn't have done it. And so many more of us are using data today than we could have done 40 years ago precisely because we have that automation that's available to us. So whenever you hear someone say, Hey, we're not gonna need to know how to code, it's just flat out wrong. You know, we need to make sure that we're building those kinds of basic proficiencies across the workforce, even if many, few of us may need to be dedicated software developers. Kaitlin LeMoine: So I feel like you're taking us in in this direction through through your last comment there, Matt, but you know, one thing we like to ask on this show, given that it's called Work Forces, is really a question about, really, what are your recommendations for how our our audience can become forces in navigating, or helping others to navigate career progression and advancement in you know what we've discussed here today as a really a shifting, ever changing landscape. Matt Sigelman:The key to that Kaitlin is in the words you just used, which is progression we tend to think about jobs, and particularly those of of our listeners who are supporting workers and learners and making effective transitions. Getting to that next job is such a, you know, often a big challenge, and it feels like a big triumph in and of itself. And I think a lot of what I would recommend is that we give more attention to the downstream consequences of each job. Where does How did to job? How do jobs connect to one another? One of the things that we've done at the Institute is we built a database of 65 million US workers career histories. Represents about 40% of the US workforce, and it's given us a very powerful way of understanding the twist, turns and tangles that run through people's careers, essentially the cow paths that that lead from one job to the next, and what it's given us a really good sense for is how much variance there is, how easy it is for people to get stuck in the one hand, but also how proximate people often are to jobs that lead to much greater opportunity. Matt Sigelman: You know, one of the things that I've often heard from people in the workforce field, and that depresses me no end, is that people with college degrees have careers and people who don't have jobs. And the reason why it upsets me so much is that the difference to me between a job and a career is that a career has a directionality to it. It has a vector. It has momentum. We recently did a report where we analyzed the careers of several million people who went straight from high school to work, and we looked at what happened to them over 20 years, and what we were able to find, what we're able to see, rather, is that there are very predictable paths to success. First of all, we found that it's true, you don't need to go to college to have a good career. We found that the top 20% of people who go straight to work after high school wind up out earning the median college graduate. But on the other hand, and we found that certain first jobs we called Launchpad Jobs, make you literally four times more likely to wind up as a top earner by the time you're 40. But we also found that there are some kinds of first jobs that make you four times more likely to wind up in or close to poverty. And here's the thing that, again, is most important to bear in mind: many of those good starts and many of the bad starts actually look the same. They pay the same and so think about what that means for somebody who's coming out of high school, they're saying, Okay, well, I could wind up seating people at a restaurant and making $23,000 a year. I can wind up as a cook in the back and make $24,000 extra, slightly more. And one of those kids, the former, will be making, if you sort of follow all the different directions that happen over their career, on average, will be making over $70,000 a year by the time they're 40, and the other one making in the low 30s, and we are providing no good insight into that. So that ability to think not just about the next job, but about what happens after that job, and what are the skills that will prepare somebody to make that next transition is, I think, one of the biggest transformations that we can we can have as as workforce professionals. Julian Alssid: Wow, Matt, it's been just incredible hearing you your download on all the these multiple aspects to what we're seeing now and and bring some clarity to the landscape. How can people follow your work? And I know you've touched on several different reports and research projects. What's you know, just as we wind down, we'd like to leave people with, you know, good, actionable info. Matt Sigelman: Well, I hope people will visit our website, Burning Glass Institute.org, all one word, and there's a place where you can sign up to join our mailing list, and we keep people abreast of the research that's coming out. Julian Alssid: Well, thank you so much for taking the time to meet with us today. This has really been a master class? Kaitlin LeMoine: Yes. Thank you so much for talking about different directions of your research. Matt Sigelman: So enjoyed this. Kaitlin LeMoine: That's all we have for you today. Thank you for listening to Work Forces. We hope that you take away nuggets that you can use in your own work. Thank you to our sponsor, Lumina Foundation. We're also grateful to our wonderful producer, Dustin Ramsdell, you can listen to future episodes at workforces dot info or on Apple, Amazon and Spotify. Please subscribe, like and share the podcast with your colleagues and friends.