Generally Intelligent
A podcast by Kanjun Qiu
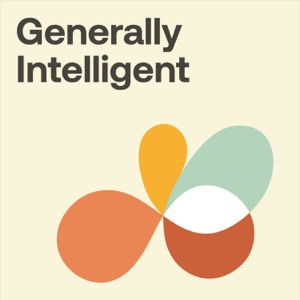
Categories:
36 Episodes
-
Episode 16: Yilun Du, MIT, on energy-based models, implicit functions, and modularity
Published: 12/21/2021 -
Episode 15: Martín Arjovsky, INRIA, on benchmarks for robustness and geometric information theory
Published: 10/15/2021 -
Episode 14: Yash Sharma, MPI-IS, on generalizability, causality, and disentanglement
Published: 9/24/2021 -
Episode 13: Jonathan Frankle, MIT, on the lottery ticket hypothesis and the science of deep learning
Published: 9/10/2021 -
Episode 12: Jacob Steinhardt, UC Berkeley, on machine learning safety, alignment and measurement
Published: 6/18/2021 -
Episode 11: Vincent Sitzmann, MIT, on neural scene representations for computer vision and more general AI
Published: 5/20/2021 -
Episode 10: Dylan Hadfield-Menell, UC Berkeley/MIT, on the value alignment problem in AI
Published: 5/12/2021 -
Episode 09: Drew Linsley, Brown, on inductive biases for vision and generalization
Published: 4/2/2021 -
Episode 08: Giancarlo Kerg, Mila, on approaching deep learning from mathematical foundations
Published: 3/27/2021 -
Episode 07: Yujia Huang, Caltech, on neuro-inspired generative models
Published: 3/18/2021 -
Episode 06: Julian Chibane, MPI-INF, on 3D reconstruction using implicit functions
Published: 3/5/2021 -
Episode 05: Katja Schwarz, MPI-IS, on GANs, implicit functions, and 3D scene understanding
Published: 2/24/2021 -
Episode 04: Joel Lehman, OpenAI, on evolution, open-endedness, and reinforcement learning
Published: 2/17/2021 -
Episode 03: Cinjon Resnick, NYU, on activity and scene understanding
Published: 2/1/2021 -
Episode 02: Sarah Jane Hong, Latent Space, on neural rendering & research process
Published: 1/7/2021 -
Episode 01: Kelvin Guu, Google AI, on language models & overlooked research problems
Published: 12/15/2020
Technical discussions with deep learning researchers who study how to build intelligence. Made for researchers, by researchers.